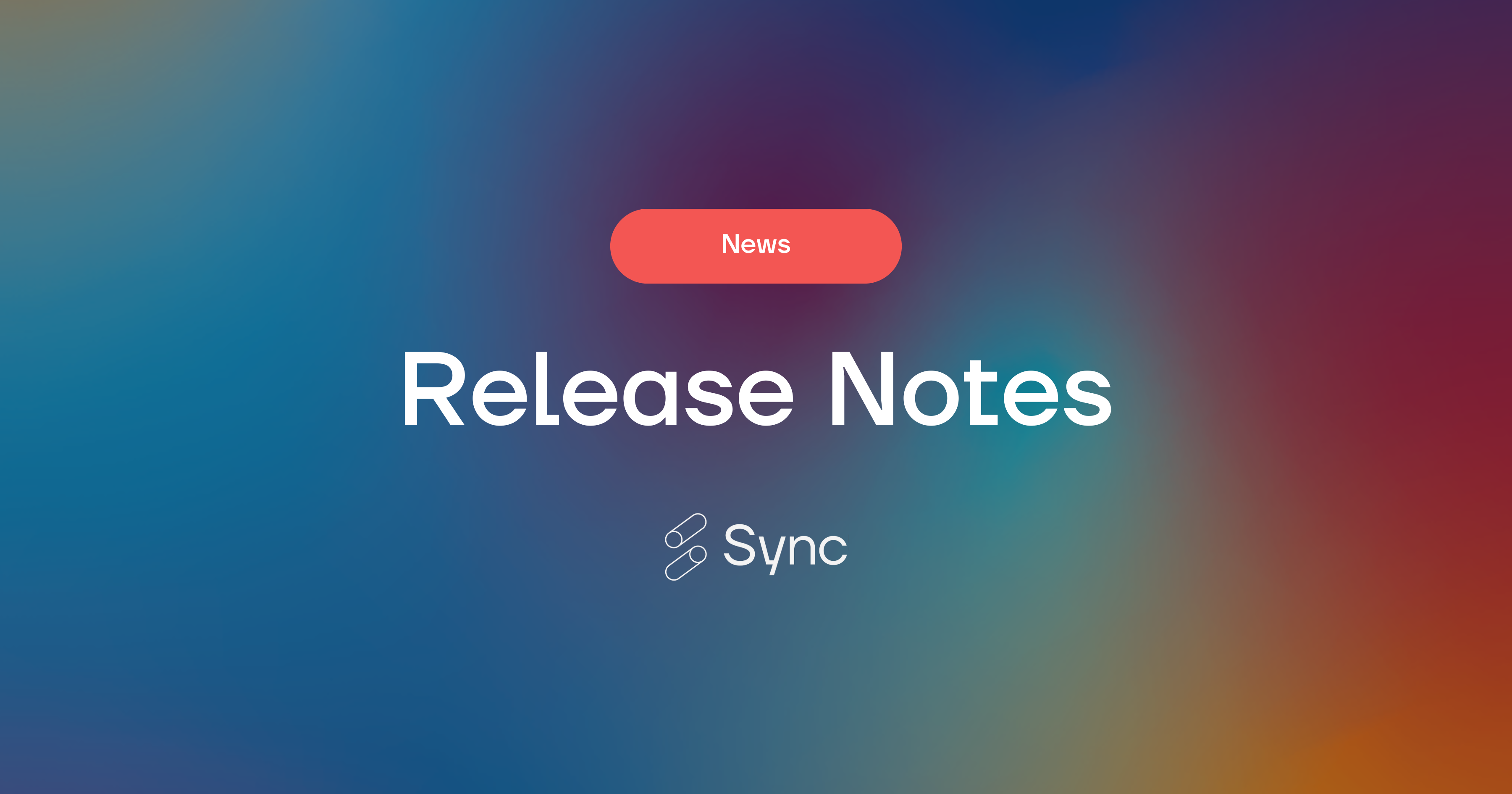
Our team has been hard at work to deliver industry-leading features to support users in achieving optimal performance within the Databricks ecosystem. Take a look at our most recent releases below.
Worker Instance Recommendations
Introducing Worker Instance Recommendations directly from the Sync UI. With this feature, you are able to tap into optimal cluster configuration recos so that your configs are optimized for individual jobs.
The instance recos within Gradient not only optimize the number of workers, but also the worker size. For example, if you are using i3.2xl instances, Gradient will find the right instance size (such as i3.xl, i3.4xl, i3.8xl, etc) in the i3 instance type.
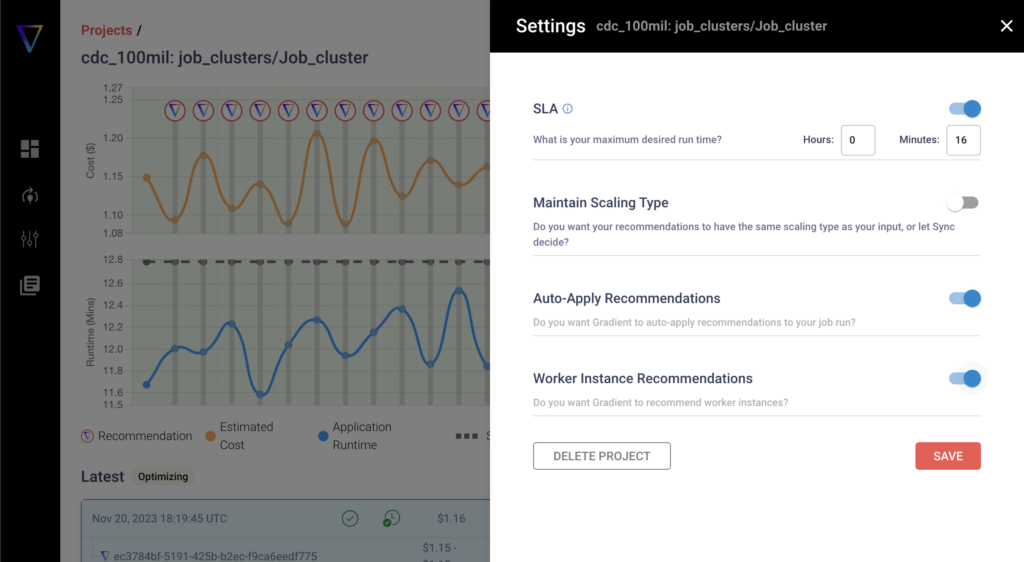
Instance Fleet Support
If your company is using Instance Fleet Clusters, Gradient is now compatible! There are no changes required on the user flow, as this feature is automatically supported in the backend. Just onboard your jobs like normal into Gradient, and we’ll handle the rest.
Hosted Log Collection
Running Gradient is now more streamlined than ever! You’re now able to opt into hosted log collection entirely in the Sync environment with Sync-hosted or user-hosted collection options. What does this mean? It means that there are no extra steps or external clusters needed to run Gradient, allowing Sync to do all the heavy lifting while minimizing the impact on your Databricks workspace.
With hosted DBX log collection within Gradient, you’re able to minimize onboarding errors due to annoying permission settings while increasing visibility into any potential collection failures, ultimately giving you and your team more control over your cluster log data.

Getting Started with Collection Setup
The Databricks Workspace integration flow is triggered when a user clicks on Add → Databricks Workspace after they have configured their workspace and webhook. Users will also now have a toggle option to choose between Sync-hosted (recommended) or User-hosted collection.
- Sync-hosted collection – The user will be optionally prompted to share their preference for cluster logs stored for their Databricks Jobs. This will initially be an immutable setting saved on the Workspace.
- For AWS – Users will need to add a generated IAM policy and IAM Role to their AWS account. The IAM policy allows us to ec2:DescribeInstances, ec2:DescribeVolumes, and optionally an s3:GetObject and s3:ListBucket to the specific bucket and prefix to which users have configured uploading cluster logs. S3 permissions are optional because they may be using DBFS to record cluster logs. The user needs to add a “Trusted Relationship” to the IAM role to give our Sync IAM role permissions to sts:AssumeRole using an ExternalId we provide them. Gradient will then generate this policy and trust relationship for the user in a JSON format to be copy and pasted.
- For Azure – Coming soon!
- For AWS – Users will need to add a generated IAM policy and IAM Role to their AWS account. The IAM policy allows us to ec2:DescribeInstances, ec2:DescribeVolumes, and optionally an s3:GetObject and s3:ListBucket to the specific bucket and prefix to which users have configured uploading cluster logs. S3 permissions are optional because they may be using DBFS to record cluster logs. The user needs to add a “Trusted Relationship” to the IAM role to give our Sync IAM role permissions to sts:AssumeRole using an ExternalId we provide them. Gradient will then generate this policy and trust relationship for the user in a JSON format to be copy and pasted.
- User-hosted collection – For both Azure/AWS will proceed as the normal workspace integration requirements dictate
Stay up to date with the latest feature releases and updates at Sync by visiting our Product Updates documentation.
Ready to start getting the most out of your Databricks job clusters? Request a demo or reach out to us at info@synccomputing.com.