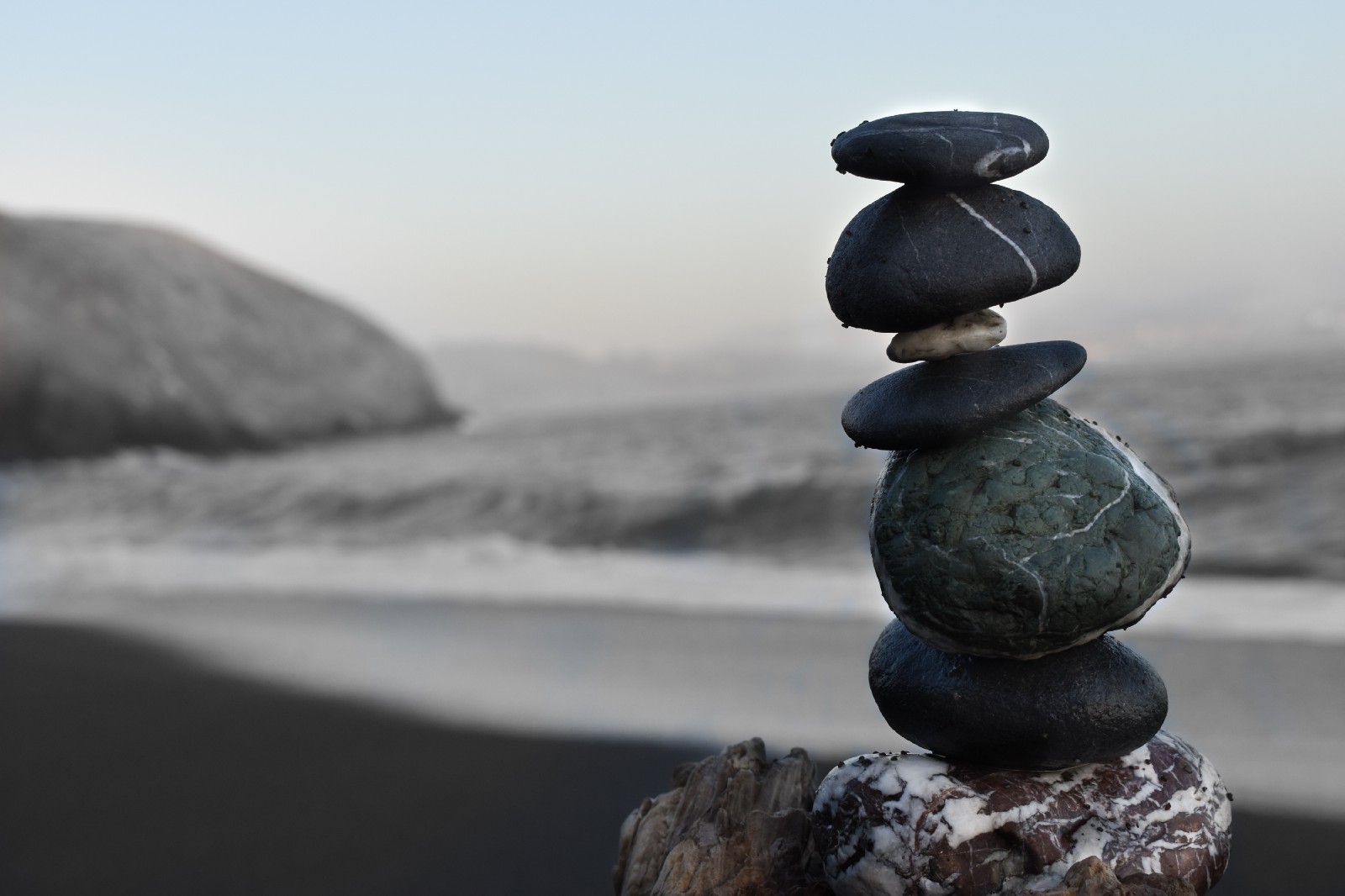
Here at Sync we are always trying to learn and optimize complex cloud infrastructure, with the goal to help more knowledge to the community. In our previous blog post we outlined a few high level strategies companies employ to squeeze out more efficiency in their cloud data platforms. One very popular response from mid-sized to large enterprise companies we hear a lot of is:
“We use Autoscaling to minimize costs”
We wanted to zoom into this statement to really understand how true it is, and to get a better understanding of the fundamental question
“Is autoscaling Apache Spark cost efficient?”
To explain in more detail, we wanted to investigate the technical side of Autoscaling and really dive deep into a specific example. Because of this we chose to begin with a gold standard workload to analyze, the TPC-DS benchmark, just to minimize any argument being made that we cherry picked a weird workload to skew the final answer. Our goal here is to be as technical and informative as possible about a few workloads – we are not trying to perform a broad comprehensive study (that would take a long time). So let’s begin:
What is Autoscaling?
For those who may not know, Autoscaling is the general concept that a cluster should automatically tune the number of workers (or instances on AWS) based on the needs of your job. The basic message told to companies is, autoscaling will optimize the cluster for your workload and minimize costs.
Technically, Autoscaling is usually a reactive algorithm that measures some utilization metric inside your cluster to determine if more or less resources are needed. While this makes logical sense, in reality the complexity of Apache Spark and constantly changing cloud infrastructure make this problem highly unpredictable.
In the Databricks UI, autoscaling is just a simple checkbox that many people may overlook. The choice people make by selecting that box could impact their overall performance significantly.
Since many people use Databricks or EMR, the exact algorithm they employ is behind closed doors, so we don’t know the exact details of their logic. The only thing we can do is measure their performance.
Experiment Setup
Our goal is to provide a technical study of Autoscaling from a novice’s point of view. Meaning, our base case to compare against will be whatever “default” settings Databricks suggests. We are not comparing against the global best or against an expert who has spent many days optimizing a particular cluster (who we think would probably do an awesome job).
- Data Platform: Databricks
- Compute type: Jobs (ephemeral cluster, 1 job per cluster)
- Photon Enabled: No
- Baseline configuration: Default params given to users at spin up
- AWS market: Driver on-demand, workers on spot with 100% on-demand fall back
- Workload: Databrick’s own benchmark on TPC-DS 100GB (all 99 queries run sequentially)
To keep things simple, we ran 3 comparison job runs:
- Fixed 8 Nodes – a fixed 8 node cluster using the default machine types suggested to us in the Databricks UI.
- Fixed 2 Nodes w/ Gradient- We use our Apache Spark Gradient product to recommend an optimized fixed custer to give us the lowest cost option (runtime not optimized). The recommendation was to use 2 nodes (with different instance types than default)
- Autoscaler 2-8 Nodes – We used the default UI settings in Databricks here.
Fixed Cluster | Fixed Cluster (Gradient) | Autoscaler 2-8 Nodes | |
No. of Workers | 8 | 2 | 2-8 |
Driver Node | i3.xlarge | r5a.large | i3.xlarge |
Worker Nodes | i3.xlarge | i3.2xlarge | i3.xlarge |
Runtime [s] | 1593 | 2441 | 2834 |
DBU Cost [$] | 0.6 | 0.39 | 0.73 |
AWS Cost [$] | 0.92 | 0.92 | 1.35 |
Total Cost [$] | 1.52 | 1.31 | 2.08 |
The results
To our surprise, of the 3 jobs run, the default autoscaler performed the worst in both runtime and cost. Both a fixed cluster of 8 nodes and 2 nodes, outperformed autoscaling in both time and cost. The Sync optimized cluster outperformed autoscaling by 37% in terms of cost and 14% in runtime.
To examine why the autoscaled cluster performed poorly, let’s look at the number of workers created and shut-down over time, in comparison to the fixed 2 node cluster. The figure below tells the basic story, that the autoscaled cluster spent a lot of time scaling up and down, tuning itself to the workload itself. At first glance, that is exactly what autoscaling is supposed to do, so why did the autoscaled cost and runtime perform so poorly?
The main reason, from what we can tell, is that there is a time penalty for changing the cluster size – specifically in upsizing the cluster. We can see from the cluster event log below, that the time between “RESIZING” and “UPSIZE_COMPLETED” can span several minutes. Based on the Spark UI, the executors don’t get launched until “UPSIZE_COMPLETED” occurs, so no new computing occurs until this step is achieved.
Another observation here is that in order for us to run the TPC-DS benchmark, we had to run an init_script to install some code at the start of the job. Based on the cluster event log below, it looks like every time it upsizes new machines, they have to reinstall all the init_scripts each time which costs time and money. This is something to consider, where if your job requires you to load specific init_scripts, this would certainly negatively impact the autoscaling performance.
So to summarize, you are paying for the “ramp up time” of new workers during autoscaling, where no computing is occurring. The more often your cluster upsizes, the more you will be waiting and paying.
Databricks mentions that using pools can help speed up autoscaling, by creating a pool of “warm” instances ready to be kicked off. Although you are not charged DBU’s, you do still have to pay AWS’s fees for those machines. So in the end, it still depends on your workload, size of cluster, and use case if the pools solution makes sense.
Another issue is the question of optimizing for throughput. If 3 nodes processes the data at the same rate as 8 nodes, then ideally autoscaling should stop at 3 nodes. But it doesn’t seem like that’s the case here, as auto-scaling just went up to the max workers set by the user.
The optimized fixed cluster looks at cost and throughput to find the best cluster, which is another reason why it is able to outperform the autoscaling solution.
Some follow up questions:
- Is this just a TPC-DS specific artifact?
We ran the same tests with two other internal Spark jobs, which we call Airline Delay and Gen Data, and observed the same trend – that the Autoscale cluster was more expensive than fixed clusters. The amount of Autoscaling fluctuation was much less for Airline delay, so we noticed the advantage of a fixed cluster was reduced. Gen Data is a very I/O intense job, and the autoscaler actually did not scale up the cluster beyond 2 nodes. For the sake of brevity, we won’t show those details here (feel free to reach out if there are more questions).
We just wanted to confirm that these results weren’t specific to TPC-DS, and if we had more time we could do a large scale test with a diverse set of workloads. Here we observed the optimized fixed cluster (using the Sync Apache Spark Gradient) achieved a 28% and 65% cost savings over default autoscaling for Airline Delay and Gen Data respectively.
- What if we just set Autoscaling to 1-2 nodes (instead of 2-8)?
We thought that if we just changed the autoscaling min and max to be near what the “Fixed 2 node gradient” cluster was, then it should get about the same runtime and cost. To our surprise, what happened was the autoscaler bounced back and forth between 1 and 2 nodes, which caused a longer job run than the fixed cluster. You can see in the plot below, we added the autoscaling job from 1-2 nodes on the worker plot. Overall the cost of the fixed 2 nodes cluster was still 12% cheaper than the autoscaled version of the same cluster with 1-2 nodes.
What this results indicates is that the parameters of min/max workers in the autoscaler are also parameters to optimize for cost and require experimentation.
- How does the cost and runtime of the job change vs. varying the autoscaling max worker count?
If the cost and runtime of your job changes based on the input into max and min worker count, then autoscaling actually becomes a new tuning parameter.
The data below shows what happens if we keep the min_worker = 2, but sweep the max_worker from 3 to 8 workers. Clearly both cost and runtime vary quite a bit compared to the Max Worker count. And the profiles of these slopes depends on the workload. The bumpiness of the total cost can be attributed to the fluctuating spot prices.
The black dashed line shows the runtime and cost performance of the optimize fixed 2 node cluster. We note that a fixed cluster was able to outperform the best optimal autoscaling configuration for cost and runtime for the TPC-DS workload.
- How did we get the cost of the jobs?
It turns out obtaining the actual cost charged for your jobs is pretty tedious and time consuming. As a quick summary, below are the steps we took to obtain the actual observed costs of each job:
- Obtain the Databricks ClusterId of each completed job. (this can be found in the cluster details of the completed job under “Automatically added tags”)
- In the Databricks console, go to the “manage account>usage tab”, filter results by tags, and search for the specific charge for each ClusterId. (one note: the cost data is only updated every couple of hours, so you can’t retrieve this information right after your run completes)
- In AWS, go to your cost explorer, filter by tags, and type in the same cluster-id to obtain the AWS costs for that job (this tag is automatically transferred to your AWS account). (Another note, AWS updates this cost data once a day, so you’ll have to wait)
- Add together your DBU and AWS EC2 costs to obtain your total job cost.
So to obtain the actual observed total cost (DBU and AWS), you have to wait around 24 hours for all of the cost data to hit their final end points. We were disappointed to see we couldn’t see the actual cost in real time.
Conclusion
In our analysis, we saw that a fixed cluster could outperform an autoscaled cluster in both runtime and costs for the 3 workloads that we looked at by 37%, 28%, and 65%. Our experiments showed that by just sticking to a fixed cluster, we eliminated all of the overhead that came with autoscaling which resulted in faster runtimes and lower costs. So ultimately, the net cost efficiency all depends on if the scaling benefits outweigh the negative overhead costs.
To be fair to the autoscaling algorithm, it’s very difficult to build a universal algorithm that reactively works for all workloads. One has to analyze the specifics of each job in order to truly optimize the cluster underneath and then still experiment to really know what’s best. This point is also not specific to Databricks, as many data platforms (EMR, Snowflake, etc) also have autoscaling policies that may work similarly.
To summarize our findings, here are a few high level takeaways:
- Autoscaling is not one size fits all – Cluster configurations is an extremely complicated topic that is highly dependent on the details of your workload. A reactive autoscaling algorithm and the overheads associated with changing the cluster is a good attempt, but does not solve the problem of cluster optimization.
- Autoscaling still requires tuning – Since Autoscaling is not a “set and forget” solution, it still requires tuning and experimentation to see what min and max worker settings are optimal for your application. Unfortunately, since the autoscaling algorithm is opaque to users, the fastest way to determine the best settings is to manually experiment.
- So when is autoscaling good to use for batch jobs? It’s difficult to provide a general answer because, like mentioned above, it’s all dependent on your workload. But perhaps two scenarios I could see are (1) if your job has long periods of idle time, then autoscaling should shut down the nodes correctly, or (2) you are running ad-hoc data science experiments and you are prioritizing productivity over costs. Scenarios (1) and (2) could be the same thing!
- So what should people do? If cost efficiency of your production level Databricks jobs is a priority, I would heavily consider performing an experiment where you select a few jobs, switch them to fixed clusters, and then extract the costs to do a before and after analysis – just like we did here.
The challenge of the last bullet is, what is the optimal fixed cluster? This is an age-old question that required a lot of manual experimentation to determine in the past, which is why we built the Apache Spark Gradient to figure that out quickly. In this study, that is how I found the optimal fixed clusters with a single file upload, without having to run numerous experiments.
Maybe autoscaling is great for your workloads, maybe it isn’t, unfortunately the answer is really “it depends.” There’s only one way to really find out – you need to experiment.