How does the worker size impact costs for Apache Spark on EMR AWS?
In this blog we analyze the impact of varying the worker instance size on cost and performance of Apache Spark in EMR using the TPC-DS 1TB benchmark.
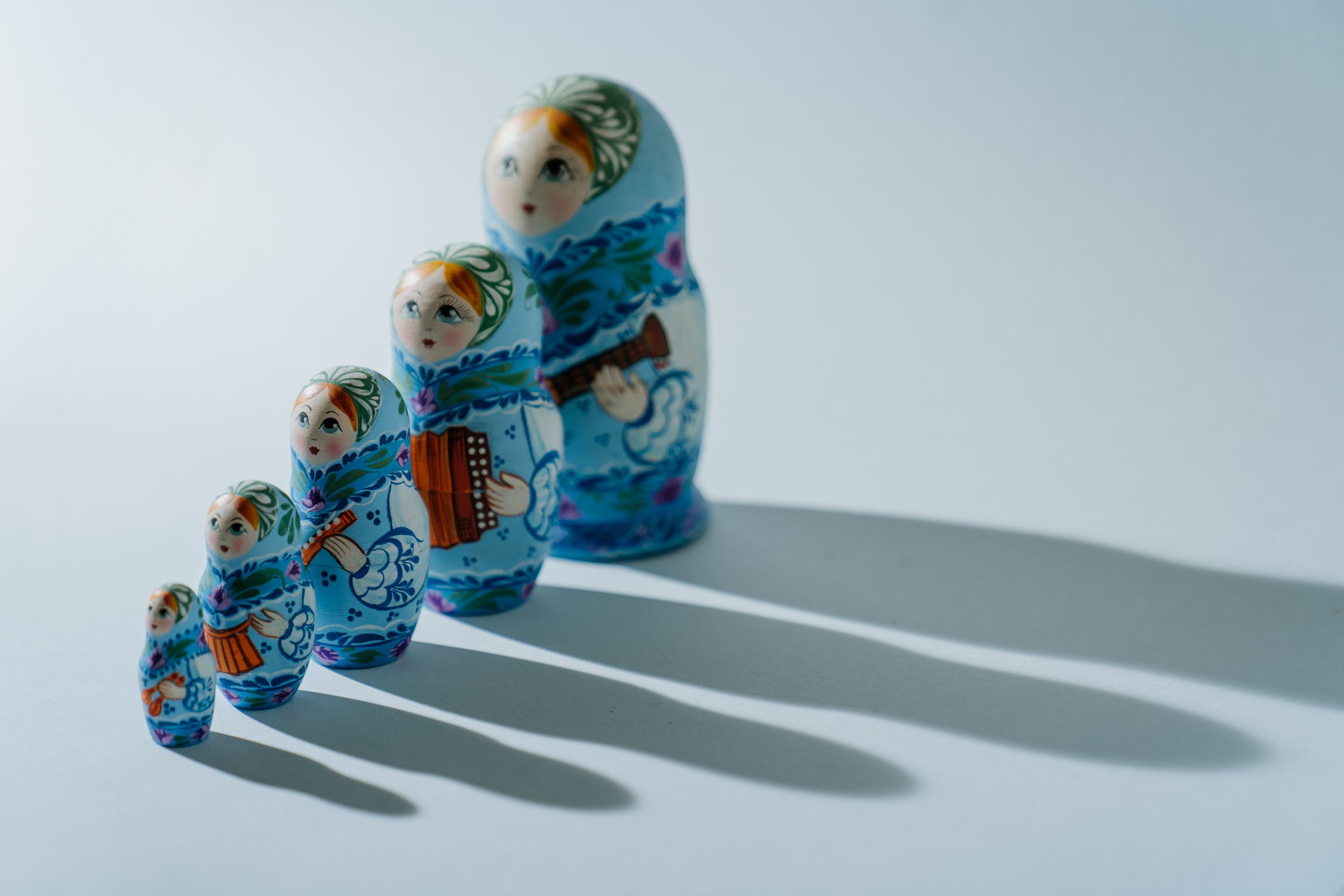
Here at Sync, we are passionate about optimizing data infrastructure on the cloud, and one common point of confusion we hear from users is what kind of worker instance size is best to use for their job?
Many companies run production data pipelines on Apache Spark in the elastic map reduce (EMR) platform on AWS. As we’ve discussed in previous blog posts, wherever you run Apache Spark, whether it be on Databricks or EMR, the infrastructure you run it on can have a huge impact on the overall cost and performance.
To make matters even more complex, the infrastructure settings can change depending on your business goals. Is there a service level agreement (SLA) time requirement? Do you have a cost target? What about both?
One of the key tuning parameters is which instance size should your workers run on? Should you use a few large nodes? Or perhaps a lot of small nodes? In this blog post, we take a deep dive into some of these questions utilizing the TPC-DS benchmark.
Before starting, we want to be clear that these results are very specific to the TPC-DS workload, while it may be nice to generalize, we fully note that we cannot predict that these trends will hold true for other workloads. We highly recommend people run their own tests to confirm. Alternatively, we built the Gradientr for Apache Spark to help accelerate this process (feel free to check it out yourself!).
With that said, let’s go!
The Experiment
The main question we seek to answer is – “How does the worker size impact cost and performance for Spark EMR jobs?” Below are the fixed parameters we used when conducting this experiment:
- EMR Version: 6.2
- Driver Node: m5.xlarge
- Driver EBS storage: 32 GB
- Worker EBS storage: 128 GB
- Worker instance family: m5
- Worker type: Core nodes only
- Workload: TPC-DS 1TB (Queries 1-98 in series)
- Cost structure: On-demand, list price (to avoid Spot node variability)
- Cost data: Extracted from the AWS cost and usage reports, includes both the EC2 fees and the EMR management fees
Fixed Spark settings:
- Spark.executor.cores: 4
- Number of executors: set to 100% cluster utilization based on the cluster size
- Spark.executor.memory: automatically set based on number of cores
The fixed Spark settings we selected were meant to mimic safe “default” settings that an average Spark user may select at first. To explain those parameters a bit more, since we are changing the worker instance size in this study, we decided to keep the number of cores per executor to be constant at 4. The other parameters such as number of executors and executor memory are automatically calculated to utilize the machines to 100%.
For example, if a machine (worker) has 16 cores, we would create 4 executors per machine (worker). If the worker has 32 cores, we would create 8 executors.
The variables we are sweeping are outlined below:
- Worker instance type: m5.xlarge, m5.2xlarge, m5.4xlarge
- Number of workers: 1-50 nodes
Results
The figure below shows the Spark runtime versus the number and type of workers. The trend here is pretty clear, in that larger clusters are in fact faster. The 4xlarge size outperformed all other cluster sizes. If speed is your goal, selecting larger workers could help. If one were to pick a best instance based on the graph below, one may draw the conclusion that:
It looks like the 4xlarge is the fastest choice
The figure below shows the true total cost versus the number and type of workers. On the cost metric, the story almost flips compared to the runtime graph above. The smallest instance usually outperformed larger instances when it came to lowering costs. For 20 or more workers, the xlarge instances were cheaper than the other two choices.
If one were to quickly look at the plot below, and look for the “lowest points” which correspond to lowest cost, one could draw a conclusion that:
It looks like the 2xlarge and xlarge instance are the lowest cost, depending on the number of workers
However, the real story comes when we merge those two plots together and simultaneously look at cost vs. runtime. In this plot, it is more desirable to be toward the bottom left, this means the run is both lower cost and faster. As the plot below shows, if one were to look at the lowest points, the conclusion to be drawn is:
It looks like 4xlarge instances are the lowest cost choice… what?
What’s going on here, is that for a given runtime, there is always a lower cost configuration with the 4xlarge instances. When you put it into that perspective, there is little to reason to use xlarge sizes as going to larger machines can get you something both faster and cheaper.
The only caveat here is there is a floor to how cheap and slow the 4xlarge cluster can give you, and that’s with a worker count of 1. Meaning, you could get a cheaper cluster with a smaller 2xlarge cluster, but the runtime becomes quite long and may be unacceptable for real-world applications.
Here’s a generally summary of how the “best worker” choice can change depending on your cost and runtime goals:
Runtime Goal | Cost Goal | Best Worker |
<20,000 seconds | Minimize | 4xlarge |
<30,000 seconds | Minimize | 2xlarge |
<A very long time | Minimize | xlarge |
A note on extracting EMR costs
Extracting the actual true costs for individual EMR jobs from the AWS billing information is not straight forward. We had to write custom scripts to scan the low level cost and usage reports, looking for specific EMR cluster tags. The exact mechanism for retrieving these costs will probably vary company to company, as different security permissions may alter the mechanics of how these costs can be extracted
If you work at a company and EMR costs are a high priority and you’d like help extracting your true EMR job level costs, feel free to reach out to us here at Sync, we’d be happy to work together.
Conclusion
The main takeaways here are the following points:
- It Depends: Selecting the “best” worker is highly dependent on both your cost and runtime goals. It’s not straightforward what the best choice is.
- It really depends: Even with cost and runtime goals set, the “best” worker will also depend on the code, the data size, the data skew, Spot instance pricing, availability to just name a few.
- Where even are the costs? Extracting the actual cost per workload is not easy in AWS, and is actually quite painful to capture both the EC2 and EMR management fees.
Of course here at Sync, we’re working on making this problem go away. This is why we built the Spark Gradient product to help users quickly understand their infrastructure choices given business needs.
Feel free to check out the Gradient yourself here!
You can also read our other blog posts here which go into other fundamental Spark infrastructure optimization questions.
More from Sync:
Databricks 101: An Introductory Guide on Navigating and Optimizing this Data Powerhouse
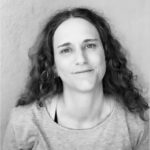
Databricks 101: An Introductory Guide on Navigating and Optimizing this Data Powerhouse
Top 9 Lessons Learned about Databricks Jobs Serverless
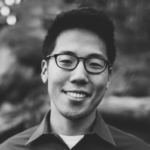
Top 9 Lessons Learned about Databricks Jobs Serverless
Gradient Product Update— Discover, Monitor, and Automate Databricks Clusters
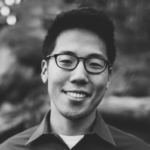
Gradient Product Update— Discover, Monitor, and Automate Databricks Clusters