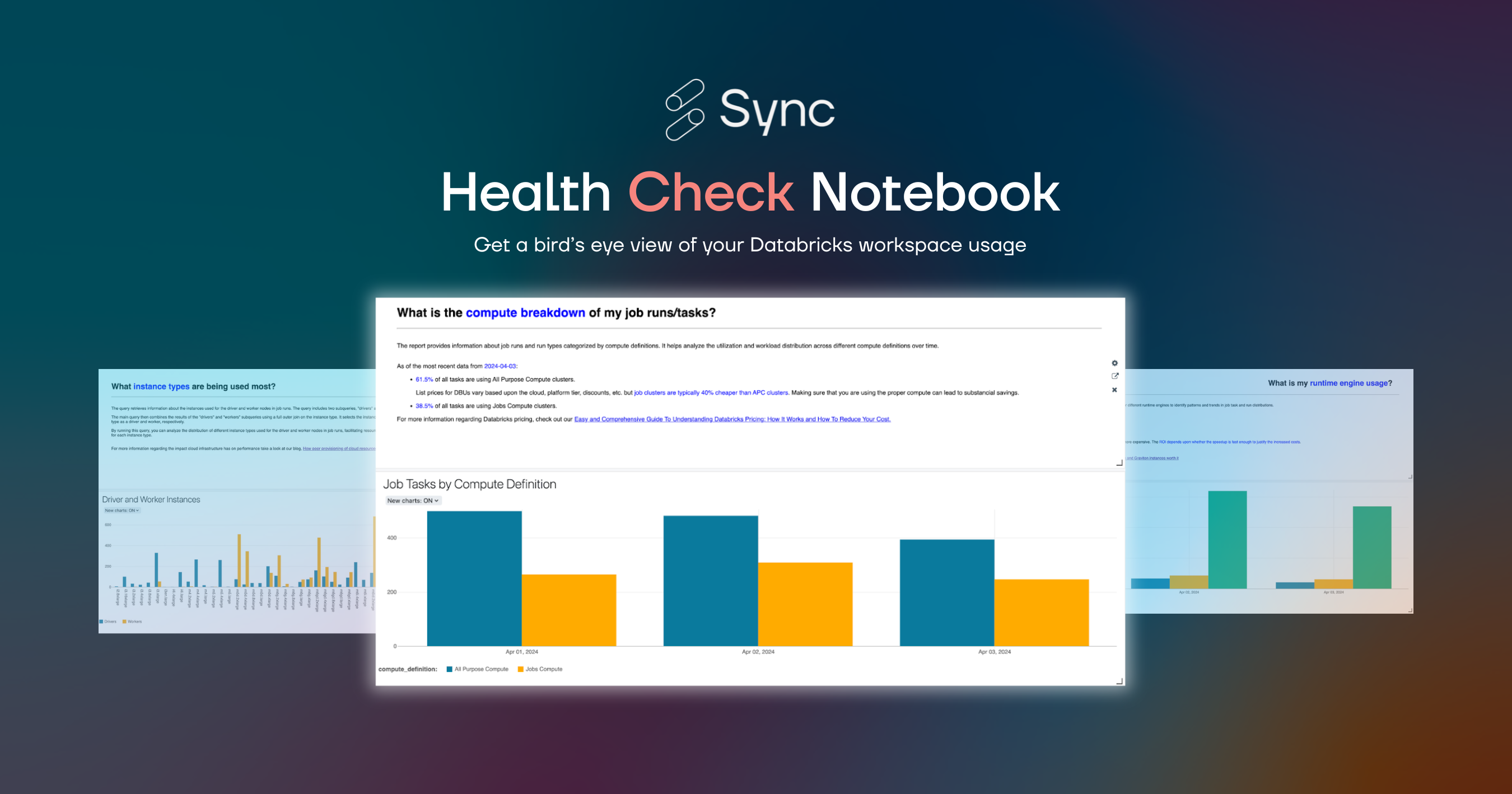
Whether you’re a data engineer, a manager of a data team, or an executive overseeing a data platform, your focus might be on growth, and to continue to build and innovate. However, this may come at the expense of ballooning costs that are getting harder and harder to get under control. This ultimately leads to a point where you need to make some tough cost-cutting decisions — like migrating to a less expensive platform — or even tougher decisions — like laying off part of your team.
Our data platform costs are increasing 20% MoM. How do we reduce our costs and get our budget under control?
Senior Data Engineer at a martech company
Can you help us get a better understanding of how we’re using Databricks? We want to get our costs under control but we don’t know where to start.
Staff Project Manager at a large pharma company
What is the Health Check?
Sync Computing’s Health Check for Databricks Workspaces is a Databricks notebook that runs entirely within your Databricks environment. It provides you with a detailed report on findings and actions that help in reducing spend, as well as lead to a deeper understanding of your use cases, patterns, and practices in Databricks.
How stable are job runs?
What is the distribution of job runs by compute type?
What does Photon usage look like?
What are the most frequently used instance types?
Are clusters being auto-terminated or sitting idle?
What are my most expensive jobs?
Sync Health Check provides answers to all the above questions, and more!
We’ll cover a few of these questions in this blog post to demonstrate how Health Check can help get you the data you need to make informed decisions when it comes to your Databricks usage.
How do I get it?
The health check for Databricks workspaces is a free tool anyone can download by following the link below:
Request health check notebook download here
We do ask for your contact information so we can follow up to see if the notebook was useful and to receive any feedback. We’d also love to hear ideas on any new analysis we can add!
Without further delay, let’s dive into what’s in the health check and how it can be a useful tool:
Job Run Stability
Jobs with low stability and failed runs cost money but don’t drive any business value. These jobs may also be preventing you from meeting your SLAs and causing thrash in your teams. Health Check shows you how many of your job runs result in success and how many result in failure. This insight helps you prioritize actions if the failures are costing you more than the business value they’re driving.
• Actionable insight: Prioritize fixing or pausing the jobs with high failure rates to save costs, deliver on SLAs, and reduce team thrash.
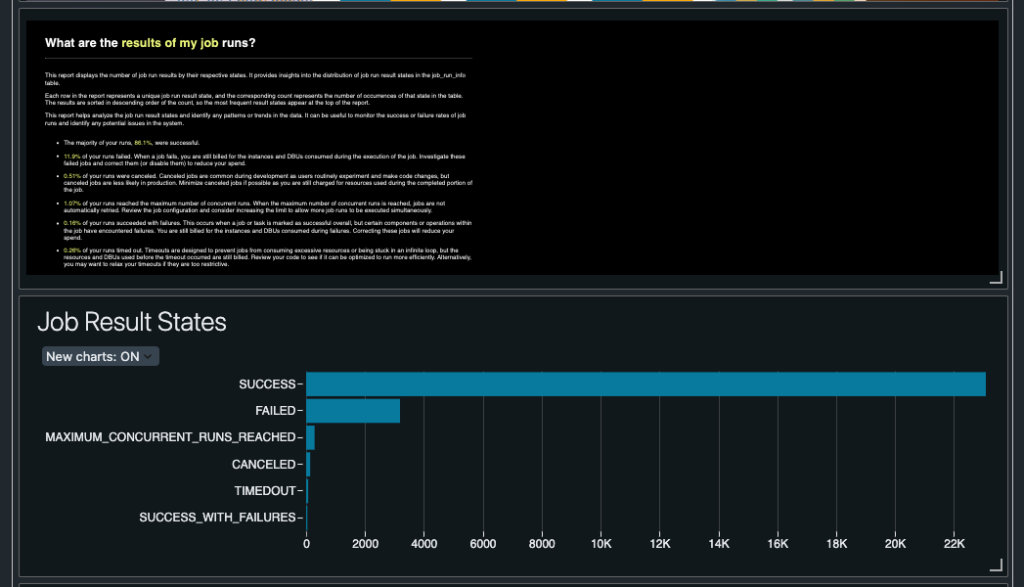
Jobs by Compute Type
Databricks offers several compute options to run your workload. For example, Jobs Compute clusters are best suited for jobs that run on a schedule while All-Purpose clusters are best suited for ad hoc analysis. We’ve seen many cases where users run scheduled jobs on All-Purpose clusters primarily to circumvent cluster spin up and spin down times. However, All-Purpose clusters come at a higher cost (at least 2.5x the cost of Jobs Compute clusters!!).
• Actionable insight: Migrate to cheaper Jobs Compute clusters and establish clear policies to grant exceptions to use All-Purpose Compute clusters.
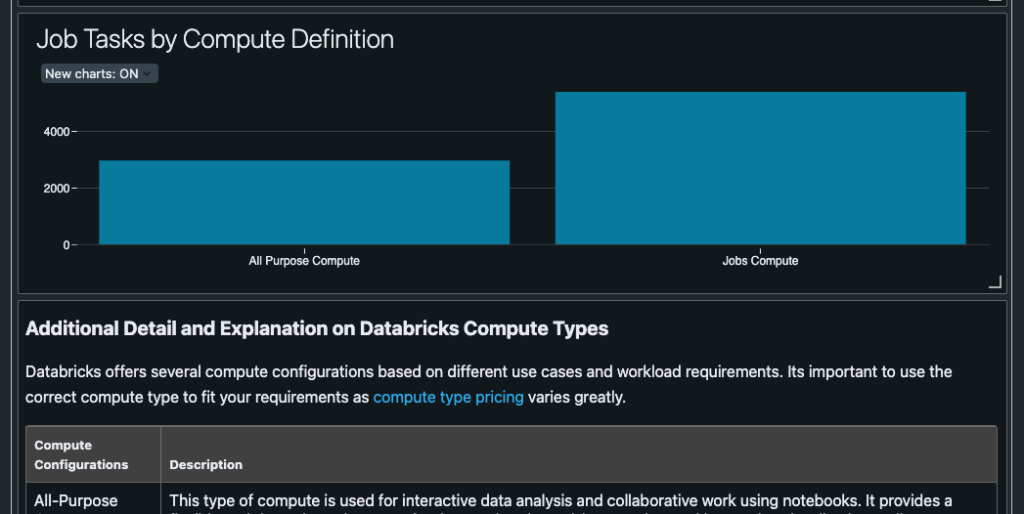
Photon Usage
Photon may do the job in terms of providing extremely fast query performance. However, whether or not it’s delivering ROI depends on the performance gain compared to the cost increase of using Photon. Note that Photon is not free and is typically a 2x cost increase for DBUs compared to non-Photon. For more information and details, check out our blog on whether Databricks clusters with Photon and Graviton instances are worth it.
• Actionable insight: Compute the ROI you’re getting out of Photon.
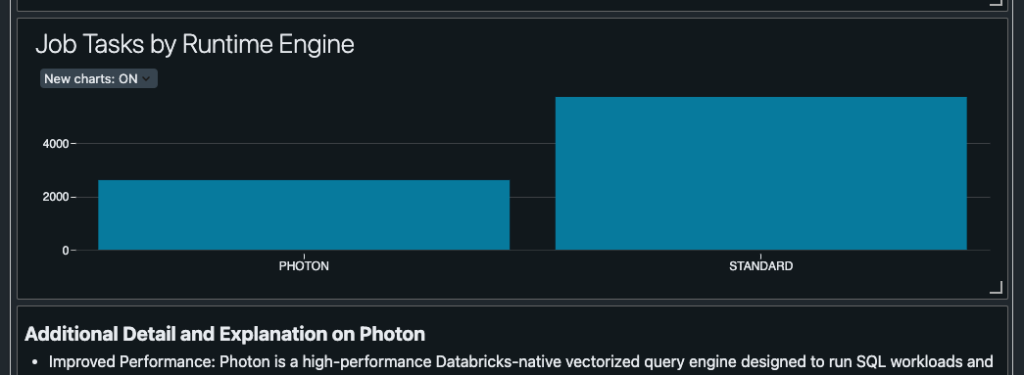
Most Frequently used Instance Types
As the subtitle suggests, this shows you the most commonly used instances. The types of instances used may change over time, as the needs of your business change. Being able to track the trends in instance types being used enables your business to remain agile and respond quickly to changing needs – such as efficiently managing your reserved instances.
• Actionable insight: Drive better alignment between Databricks instance usage and your organization’s preferred instances.
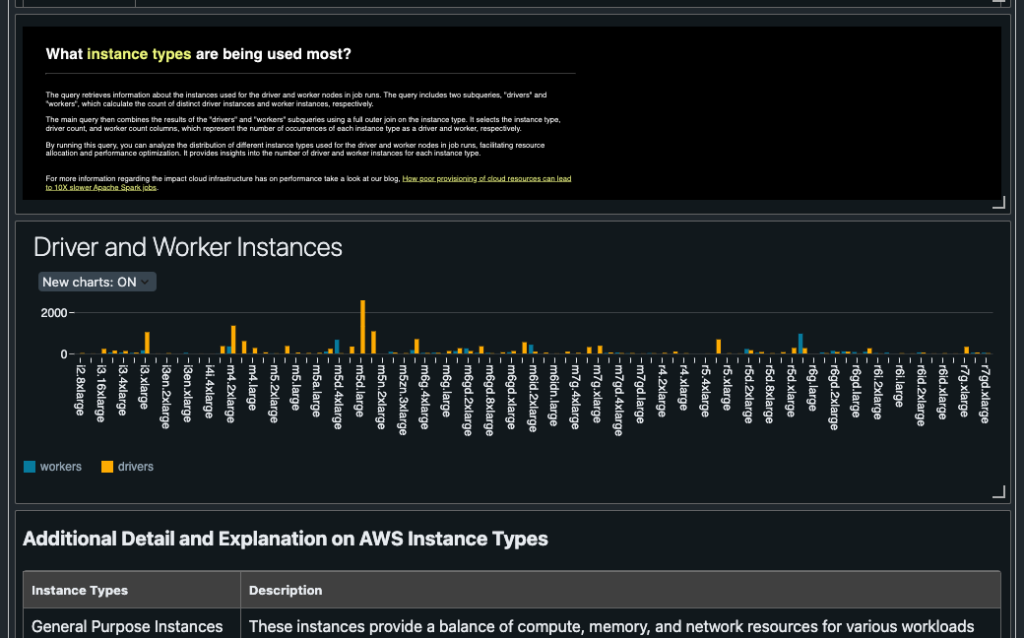
Auto-Termination
Clusters with no auto-termination, or longer auto-termination continue to accrue costs when they’re idle. This is generally the case with All-Purpose compute clusters, and the wasted spend could have been avoided with better policies on auto-termination. Additionally, with more of these clusters being spun up, the total idle time keeps increasing. Jobs Compute clusters, on the other hand, are terminated right after the job completes so there’s generally no waste related to idle time.
• Actionable insight: Set auto-termination to a minimum for your clusters and establish clear policies to grant exceptions while encouraging cluster re-use.
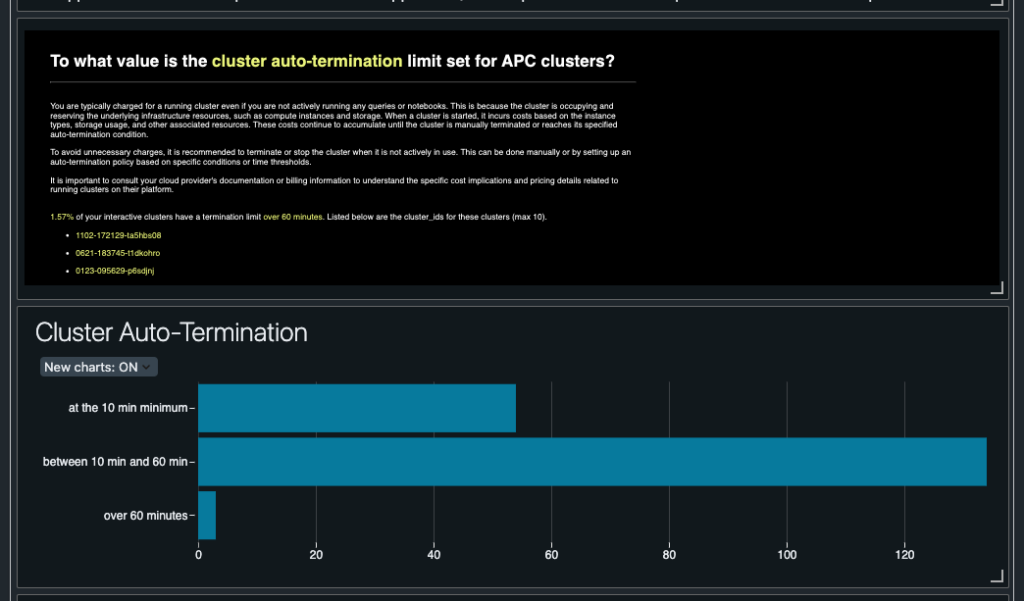
Most Expensive Jobs
Health Check shows you your top most expensive jobs based on DBUs alone. This is only part of the picture, but when compared against the business value that these jobs drive then you can determine whether you’re getting ROI out of these jobs. If you’ve determined that the job is high value, then the next step is to increase ROI through rightsizing. A major cause of bloated costs is over-provisioning, where you’re still paying for underutilized resources.
• Actionable insight: Determine if these jobs are high value and whether there’s opportunity to rightsize the compute to move the needle on ROI.
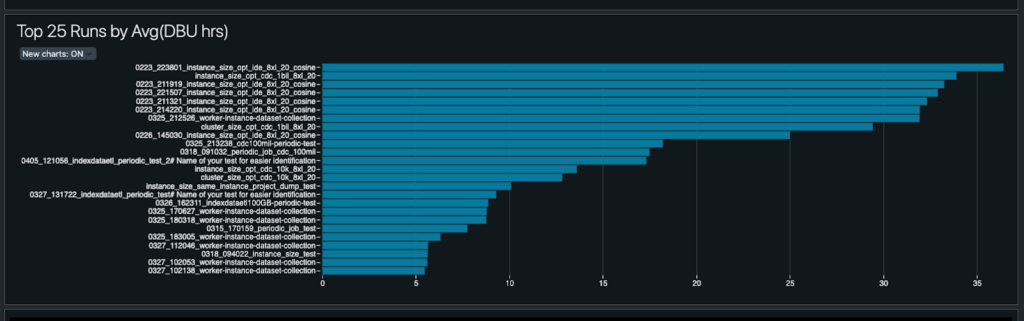
Wrapping Up
Sync’s Health Check provides deep insights into how your organization uses Databricks, and shines light on areas where there is opportunity to improve.
Feel free to reach out to us! We’d love to hear your feedback on how the Sync Health Check worked for you, and where there’s room for improvement. You can reach us here or send an email to our support team.