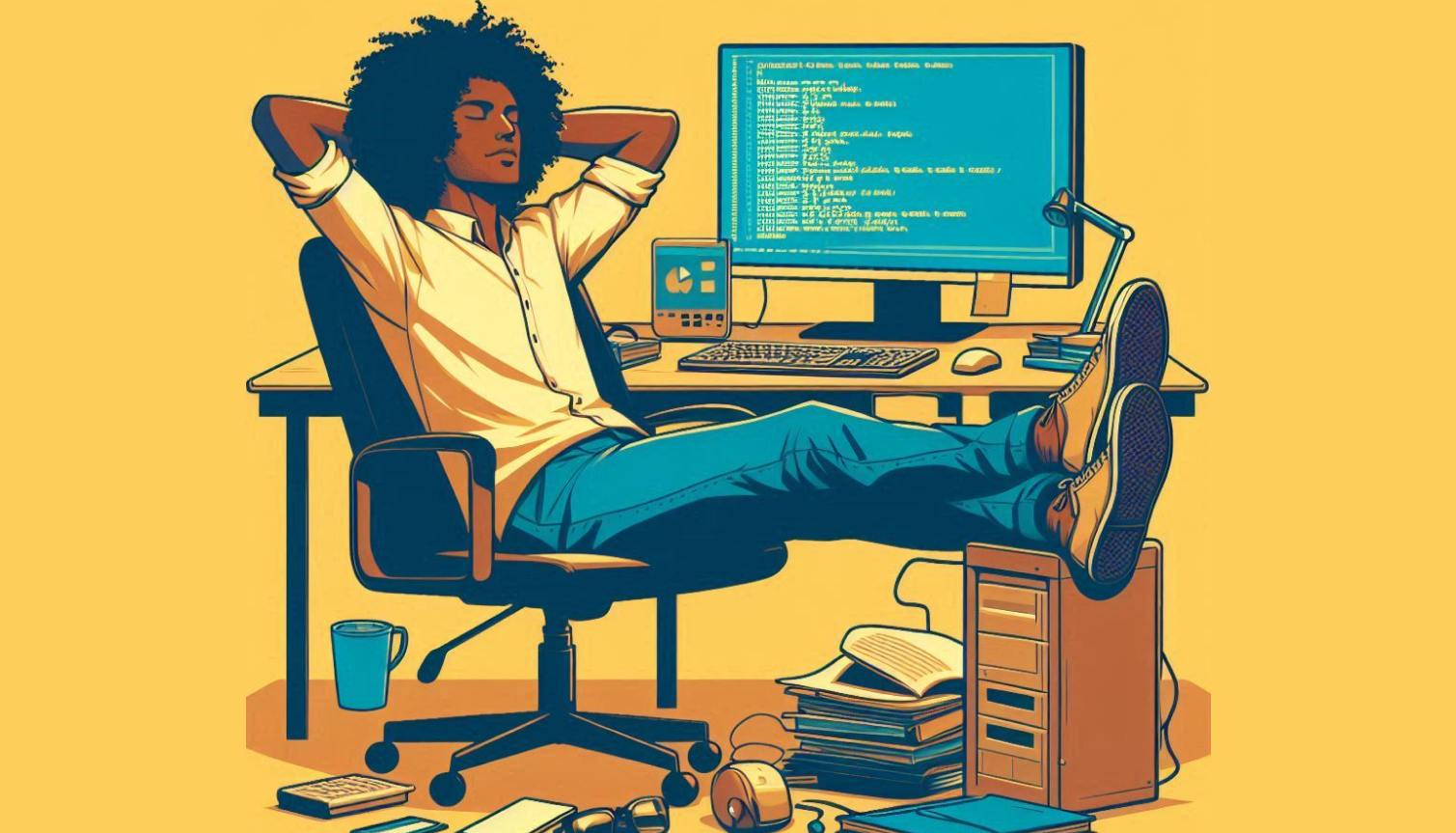
In an era where data reigns supreme, the tools and platforms that businesses utilize to harness and analyze their data can make or break their competitive edge. Among these, Databricks stands out as a powerhouse, yet navigating its complexities often feels like deciphering cryptic code. With businesses generating an average of 2.5 quintillion bytes of data daily, the need for a robust, efficient, and cost-effective data cloud has never been more critical.
In this post, we demystify Databricks, with a focus on Job Clusters. Readers will gain insight into the platform’s workspace, the pivotal role of workflows, and the nuanced world of compute resources including All-Purpose Compute (APC) clusters vs Jobs Compute clusters. We’ll also shed light on how to avoid costly errors and optimize resource allocation for data workloads.
Curious about how Databricks can transform your data strategy while keeping costs in check? Read on to learn how.
Introduction to Databricks: Components and configurations

Databricks, at its core, is a comprehensive platform that integrates various facets of data science and engineering, offering a seamless experience for managing and analyzing vast datasets.
Central to its ecosystem is the Workspace, a collaborative environment where data teams can store files, collaborate on notebooks, and orchestrate data workflows, to name a few capabilities. Workspaces act as the nerve center along with the Unity Catalog, which provides a bridge between workspaces. Together they facilitate an organized approach to data projects and ensure that valuable insights are never siloed.
Workflows, available under each Workspace, represent recurring production workloads (aka jobs) which are vital for database production environments. These workflows automate routine data processing tasks, such as machine-learning pipelines, ETL workloads, and data streaming, ensuring reliability and efficiency in data operations. Understanding the significance of workflows is essential for anyone looking to streamline their data processes in Databricks.
Databricks Job Clusters
Workflows rely on Job Clusters for compute resources. Databricks offers various compute resource options to pick from during the cluster set up process. While the default cluster compute is set to Serverless, you can expose and tweak granular configuration options by picking one of the classic compute options.
Read on for more info about Databricks Job Cluster options.
Serverless Jobs vs. Classic Compute
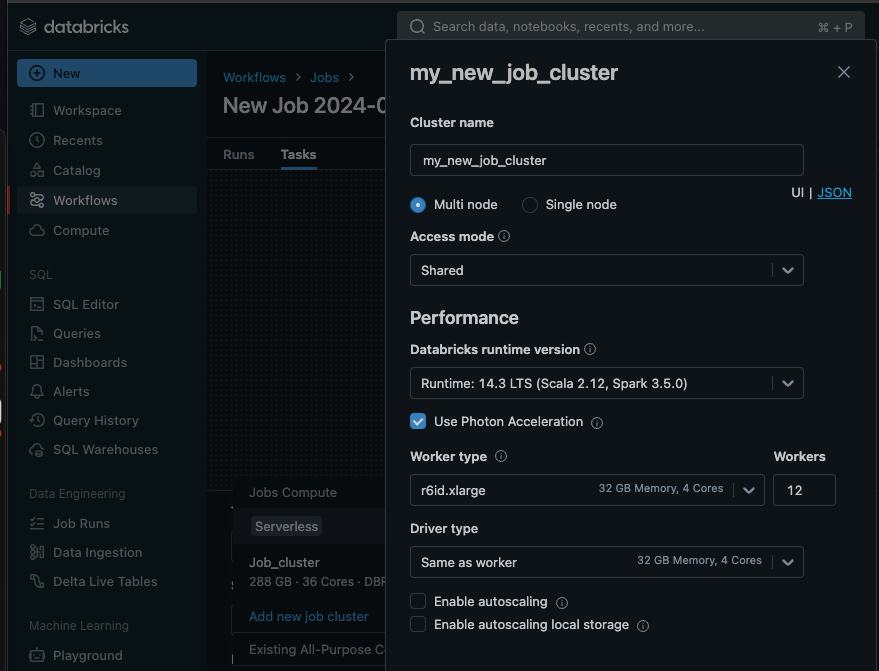
Databricks recently announced the release of serverless compute across the platforms at the Data + AI 2024 conference. The goal is to provide users with a simplified cluster management experience and reduce compute costs. However, based on our research, Jobs Serverless isn’t globally cheaper than Classic Compute, and there is a price for the convenience that serverless offers.
We compared the cost and performance of serverless jobs vs jobs running on classic clusters and found that while short ad-hoc jobs are ideal candidates for serverless, optimized classic clusters outperformed their serverless counterparts by 60% in costs.
More about the lessons we learned from experimenting with Databricks Serverless Jobs.
As for the convenience aspect, it does come at a cost. Serverless compute unburdens you from setting up cluster configuration, but that also means you lose control over job performance and costs. There are no settings to tweak or prices to negotiate with cloud providers. So if you are like us, and want to be able to control the configuration of your data infrastructure this might not be the right option for you.
On-demand clusters vs. Spot instances
Compute resources form the backbone of Databricks cluster management. The basic classification of compute resources is between on-demand clusters vs Spot instances. Spot instances are considered cost effective, offering discounts of up to 90% on remnant cloud compute. However, they aren’t the most stable or reliable. This is because the number of workers running can change in the middle of a job, which is dangerous. The job runtime and cost could become highly variable, and sometimes even crash.
Overall, on-demand instances are better suited for workloads that cannot be interrupted, workloads with unknown compute requirements, and immediate launch and operation needs. Spot instances, on the other hand, are ideal for workloads that can be interrupted and stateful applications with surge usage.
Spot vs On-demand | On-demand Instances | Spot Instances |
Access | Immediate | Only if there is unused compute capacity |
Performance | Stable and reliable | Limited stability as workers can be pulled during a job run |
Cost | Known | Varies. Up to 90% cheaper than on-demand rates |
Best for | Jobs that cannot be interrupted Jobs with unknown compute requirements | Jobs that can be interrupted Stateful apps with surge usages |
All-Purpose Compute vs Jobs Compute
Databricks offers a couple forms of compute resources, including All-Purpose Compute (APC) clusters, Jobs Compute clusters, and SQL warehouses. Each resource is designed with specific use cases in mind.
SQL warehouses, for example, allow users to query information in Databricks using the SQL programming language. APCs and Jobs Compute, on the other hand, are more general compute resources capable of running many different languages such as Python, SQL, and Scala to run your jobs. While APCs are ideal for interactive analysis and collaborative research, Jobs Compute is ideal for scheduled jobs and workflows. Understanding the distinctions and use cases for these compute resources is crucial for optimizing performance and managing costs.
Unfortunately, navigating the compute options for your clusters can be difficult. Common errors include the accidental deployment of more expensive compute resources, such as APC cluster when a Jobs Compute cluster would suffice. Such mistakes can have significant financial implications. In fact, we’ve found that APCs can cost up to 50% more than running the same job using Jobs Compute.
The difference between APC clusters and Jobs Compute clusters represents a crucial decision point for Databricks users, as they spin up a cluster. And each option is tailored to different stages of the data analysis lifecycle. Knowing these nuances can help you avoid common errors to ensure that your Databricks environment is both effective and cost-efficient. For example, APC clusters can be used for more exploratory research work while Job Clusters are used for mature production scheduled workloads.
Photon workload acceleration
Databricks Photon is a high-performance vectorized query engine that accelerates workloads.
Photon can substantially speed up job execution, particularly for SQL-based jobs and large-scale production Spark jobs. Unfortunately, Photon costs about 2x/DBU.
Learn more about the pros and cons of Databricks Photon in this post.
Many factors influence whether Photon is the right choice for you, which is why we recommend you A/B test the same job with and without Photon enabled. From our experience, 9 out of 10 organizations opt out of Photon once the results are in.
Spark autoscaling
Databricks offers an autoscaling feature to dynamically adjust the number of worker nodes in a cluster based on the workload demands.
By dynamically adjusting resources, autoscaling can reduce costs for some jobs. However, due to the spin up time cost of new workers, sometimes autoscaling can lead to increased costs. In fact, we found that simply applying autoscale across the board can lead to a 30% increase in compute costs.
Read the full analysis of Databricks autoscaling here.
Notebooks for ad-hoc research in Databricks
Databricks revolutionizes the data science and data engineering landscape with its notebook concept, which combines code with annotations. It embodies a dynamic canvas where you can breathe life into your projects.
Notebooks in Databricks excel in facilitating chunk-based code execution, a feature that significantly enhances debugging efforts and iterative development. By enabling users to execute code in segments, we eliminate the need for running entire scripts for minor adjustments. This accelerates the development cycle and promotes a more efficient debugging process.
Notebooks can run on APC clusters and Jobs Compute clusters depending on the task at hand. This choice is paramount when project requirements dictate the need for either exploratory analysis when APC is ideal, or scheduled jobs when Jobs Compute will be the most cost effective.
It’s important to note that All-Purpose Compute clusters are shared environments with a collaborative aspect. Multiple data scientists can simultaneously work on the same project with collaboration facilitated by shared compute resources. As a result, team members can contribute to and iterate on analyses in real-time. Such a shared environment streamlines research efforts, making APC clusters invaluable for projects requiring collective input and data exploration.
The journey from ad-hoc research in notebooks to the production-ready status of workflows marks a significant transition. While notebooks serve as a sandbox for exploration and development, workflows embody the structured, repeatable processes essential for production environments.
This contrast underscores the evolutionary path from exploratory analysis, where ideas and hypotheses are tested and refined, to the deployment of automated, scheduled jobs that operationalize the insights gained. It is this transition from the “playground” of notebooks to the “factory floor” of workflows that encapsulates the full spectrum of data processing within Databricks, bridging the gap between initial discovery and operational efficiency.
From development to production: Workflows and jobs
The journey from ad-hoc research in notebooks to the deployment of production-grade workflows in Databricks encapsulates a sophisticated transition, pivotal for turning exploratory analyses into automated, recurring processes that drive business operations. At the core of this transition are workflows, which serve as the backbone for scheduling and automating jobs in Databricks.
Workflows stand out by their ability to convert manual, repetitive tasks into automated sequences that run based on predefined triggers. These triggers vary widely, from the arrival of new files in a data lake to continuous data streams that require real-time processing. By leveraging workflows, data teams can ensure that their data pipelines are responsive, timely, and efficient, enabling seamless transitions from data ingestion to insight generation.
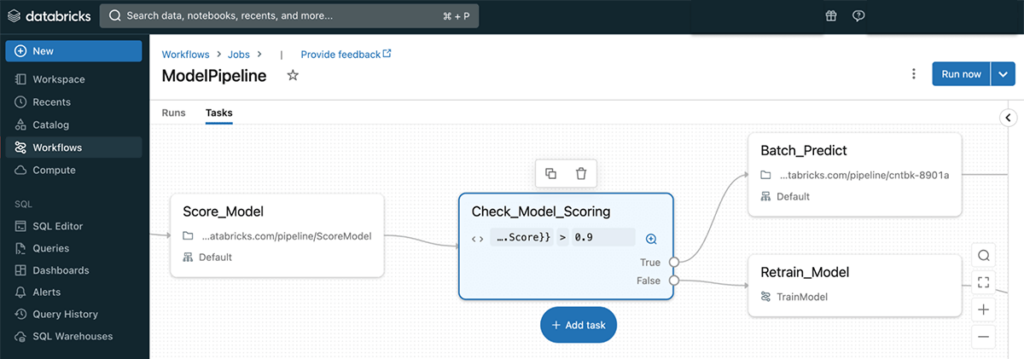
Directed Acyclic Graphs (DAGs) are central to workflows and further enhance the platform by providing a graphical representation of workflows. DAGs in Databricks allow users to visualize the sequence and dependencies of jobs, offering a clear overview of how data moves through various processing stages. This is an essential feature for optimizing and troubleshooting workflows, enabling data teams to identify bottlenecks or inefficiencies within their pipelines.
Transitioning data workloads from the exploratory realm of notebooks to the structured environment of production-grade workflows enables organizations to harness the full potential of their data, transforming raw information into actionable insights.
Orchestration and Competition: Databricks Workflows vs. Airflow and Azure Data Factory
By offering Workflows as a native orchestration tool, Databricks aims to simplify the data pipeline process, making it more accessible and manageable for its users. With that said, it also opens up a complex landscape of competition, especially when viewed against established orchestration tools like Apache Airflow and Azure Data Factory.
Comparing Databricks Workflows with Apache Airflow and Azure Data Factory reveals a compelling competitive landscape. Apache Airflow, with its open-source lineage, offers extensive customization and flexibility, drawing users who prefer a hands-on, code-centric approach to orchestration. Azure Data Factory, on the other hand, integrates deeply with other Azure services, providing a seamless experience for users already entrenched in the Microsoft ecosystem.
Databricks Workflows promise simplicity and integration, especially appealing to those who already leverage the platform. The key differentiation lies in Databricks’ ability to offer a cohesive experience, from data ingestion to analytics, within a single platform.
The strategic implications for Databricks are multifaceted. On one hand, introducing Workflows as an all-in-one solution locks users into its ecosystem. On the other hand, it challenges Databricks to continually add value beyond what users can achieve with other tools. The delicate balance between locking users in and providing unmatched value is critical; users will tolerate a certain degree of lock-in if they perceive they are receiving significant value in return.
As Databricks continues to expand its suite of tools with Workflows and beyond, the landscape of data engineering and analytics tools is set for a dynamic evolution. The balance between ecosystem lock-in and value provision will remain a critical factor in determining user adoption and loyalty. The competition between integrated solutions and specialized tools will shape the future of data orchestration, with opportunities for both consolidation and innovation.
Conclusion
In today’s data-driven world, mastering tools like Databricks is crucial. This guide aims to help simplify the management of Databricks Job Clusters. Our goal is to help you navigate the complexities and optimize resource allocation effectively.
Understanding the distinction between compute resources, such as APC clusters for interactive analysis and Jobs Compute clusters for scheduled jobs, is essential. Understanding when to apply Photon and when not to is also critical for cluster optimization. Simply choosing the right compute options for your jobs can reduce your Databricks bill by 30% or more.
The journey from exploration and research in notebooks to production-ready automated and scheduled workflows is a significant evolution. Workflows simplify data pipeline orchestration, and compete with other data orchestration tools like Apache Airflow and Azure Data Factory.
While Airflow offers extensive customization and Azure Data Factory integrates seamlessly with Microsoft services, Databricks Workflows provide a cohesive experience from data ingestion to analytics. The challenge for Databricks is to balance ecosystem lock-in with delivering unmatched value, ensuring it continues to enhance its tools and maintain user loyalty in an evolving data landscape.
If you are interested in learning more about Databricks cluster management and cost optimization follow us on Medium, or subscribe to your newsletter using the form in our footer.
If you’ve already ramped up your activity on Databricks and are looking for a way to cut costs, we can help you reduce spend by 50%. Use this free Databricks workspace health check for an estimation of your potential savings, and actionable insights into your cluster configurations (most expensive jobs, candidates for Photon and autoscaling, APC mistakes, and more).