AdTech company saves 300 eng hours, meets SLAs, and saves $10K with Gradient
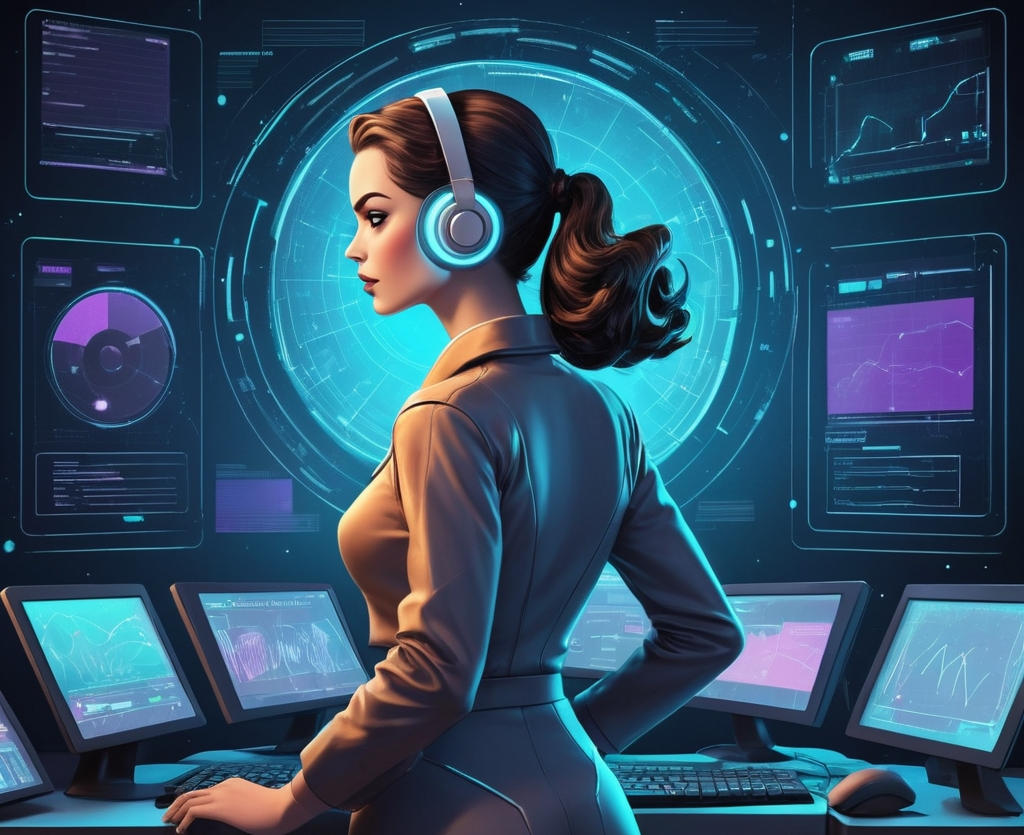
Today, balancing performance requirements with cost efficiency is a critical challenge for both data engineers and executives. A leading advertising analytics platform faced this significant challenge. With strict customer-facing runtime Service Level Agreements (SLAs) to meet efficiently, the company needed a solution that could optimize their data infrastructure for both performance and compute costs.
This is what compelled the AdTech company to explore Gradient by Sync, an AI compute optimization engine. The company focused Gradient on optimizing three key jobs in production.
Here are the key results for three key jobs:
- 2 out of 3 jobs met their runtime SLAs +90% of the time at lower costs.
- $10K/year projected savings from just these three jobs
- +300 engineering hours saved with automated optimization
These results demonstrate Gradient’s ability to optimize data infrastructure for efficiency and cost-effectiveness. The company runs hundreds of jobs, while this post is only focuses on three key jobs. Read on for all the details.
The results
Gradient uses advanced, self-improving machine-learning algorithms to recommend optimal compute configurations based on specific business goals, such as cost savings or meeting runtime SLAs. The resulting recommendations can be reviewed by your team and applied with a single click, or automated for optimization at scale.
The optimization techniques utilized are unique per workload, but they all aim to maximize efficiency and minimize costs. Depending on workload characteristics, the optimization can result in cost savings, performance improvements, or both.
By default, Gradient is set to reduce costs. But if you input your desired runtime SLAs, Gradient will optimize your clusters to hit that SLA at the lowest possible price point. This case-study is a great real-world example.
Job 1: 92% SLA match rate and -33% cost/GB
This job was initially set to target a runtime of 25 mins. With this constraint in place, Gradient was able to ensure the SLA was met for at least 9 out of 10 runs.
When the runtime SLA was increased to 28 minutes, Gradient was able to ensure the new target was met consistently and reduce the compute cost of this workload. In fact, the average job run cost was cut by 13%, and the company is projected to save $5K a year from this job alone.
A deeper look into this workload reveals an interesting finding. The average data size for this job grew by 29%. While not uncommon by any means, data size fluctuations tend to impact both performance and costs. So it’s interesting to see that the average cost per run was actually reduced by 13%.
This is where the average cost/GB metric can help. This normalized metric helps assess the efficiency of your data pipelines, as they grow and evolve. In the summary table above, you can see that while the average data size grew from an average 57GB to 73.4GB per run, the average cost per gigabyte processed fell from $0.036 to $0.024. This 33% reduction in compute cost per gigabyte has resulted in a 13% decrease in average cost per run.
We believe that the cost/GB metric can reduce much of the complexity around assessing ROI from your data optimization projects.
Go here for more about the cost/GB metric.
Job 2: 98.6% SLA met with 8% cost savings
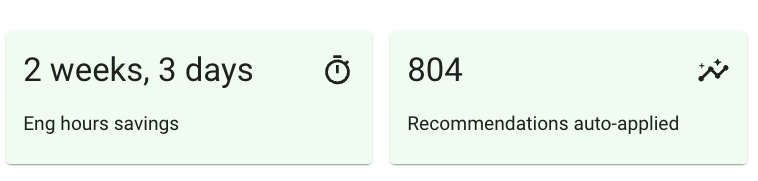
This job experienced a significant runtime reduction, likely due to changes in data size and/or code made by the AdTech company. Gradient’s adaptive algorithm responded by reducing the cluster size and was able to reduce costs and hit the runtime SLA. This optimization resulted in cost savings, without compromising performance. In fact, Gradient was able to drive a 8% cost reduction compared to the rate directly after the runtime reduction. This optimization is projected to save the company about $3K a year.
This is a stellar example of the many factors that can impact the performance and costs of your data pipelines. The company was able to significantly reduce runtimes with data and code optimization. Gradient automatically responded by gradually reducing the number of cluster resources until it reached the minimal cost in which it can meet the desired runtime.
It took Gradient just north of 800 optimizations to reach that point. Based on our calculation, it would take the company a minimum of 100 hours of engineering time to attempt to manually tune the clusters to get the same result.
By gradually optimizing the compute configuration, Gradient ensures the optimizations it performs are statistically significant, so that companies see long term cost savings. This is in stark contrast to manual tuning, where we might see improvements one day, but the next day a change in data size will make everything obsolete.
Job 3: 30% faster runtimes and $3K cheaper
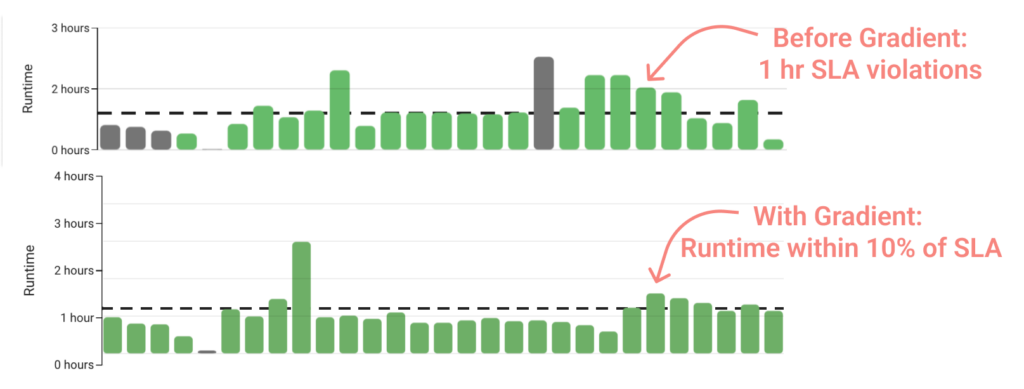
This job presented the most significant challenge. Its data size would vary, with “large” data sizes causing runtimes to by far exceed the SLA target. Gradient was able to speed up the runtimes of “large” data sizes by a whopping 30% by dynamically changing the cluster resource allocation based on projected data size.
Ultimately, Gradient was able to find the optimal configuration to meet every “small” data sized run, and most “large” ones. As a result, the company is projected to save $3K a year on this job. While not fully meeting the SLA target, the job is much closer to it and is running at a lowered cost, as per the company’s objectives.
Business impact
The company’s objective is to meet SLAs at a reasonable cost. To this end, they seek evidence for each of Gradient’s core capabilities: cost savings, performance improvements, and increased engineer productivity.
Improved performance
Two out of three jobs optimized hit their runtime SLAs +90% of the time, and at a lower rate. The third job saw a 30% improvement, bringing it significantly closer to its SLA target and driving thousands of dollars of savings a year.
Cost savings
Gradient drove cost savings across all three jobs, even when the primary goal was to meet SLAs. This dual benefit of improved performance and reduced costs highlights the advanced ML models that drive these recommendations. Models that are automatically fine-tuned on your jobs to offer 100% custom recommendations based on the unique characteristics of your data pipelines.
Increased productivity
Gradient applied more than 2,300 optimizations. This automated approach to cluster tuning saved the advertising analytics company an estimated +300 engineering hours that would have otherwise been spent manually adjusting cluster configurations to meet runtime SLAs.
Summary of results
Job | Cost savings | Projected annual savings | Recommendations applied | Runtime SLA match rate |
1 | 13% | $4,573 | 827 | 92% |
2 | 8% | $2,920 | 804 | 98.6% |
3 | 3.6% | $3,022 | 695 | 75% |
How does Gradient manage compute?
Gradient dynamically re-configures compute resources based on company goals (e.g. cost savings, or runtime SLAs) and workload demands (e.g. variable sized-data). It continuously monitors your job to seamlessly adapt and alert you of any anomalies or workload changes. Each job is paired with one of our advanced ML models which is fine-tuned on that job to offer customized optimizations for that workload based on its unique characteristics.
For more information go here, or check out our most detailed explanation of how our self-improving ML models work.
Conclusion
A leading AdTech company was faced with the all too common challenge of balancing costs with performance. By leveraging Gradient’s advanced optimization capabilities, the company was able to meet runtime SLAs for critical jobs, which was their primary goal. They were also able to achieve substantial cost savings, improve workload performance (even for challenging workloads), and regain more than 300 engineering hours for more strategic tasks.
As the company continues to work with Gradient, they are well-positioned to further enhance their data operations, maintain their competitive edge, and scale efficiently in the media intelligence industry.
We’ve helped them and we can do the same for you! If you’re ready to start saving today, run our free Databricks workspace healthcheck for instant insights in your data infrastructure, or book a personalized demo.
More from Sync:
Adding an AI agent to your data infrastructure in 2025
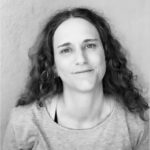
Adding an AI agent to your data infrastructure in 2025
Choosing the right Databricks cluster: Spot instances vs. on-demand clusters, All-Purpose Compute vs. Jobs Compute
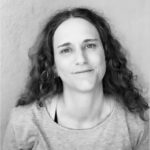
Choosing the right Databricks cluster: Spot instances vs. on-demand clusters, All-Purpose Compute vs. Jobs Compute
Databricks Compute Comparison: Classic Jobs vs Serverless Jobs vs SQL Warehouses
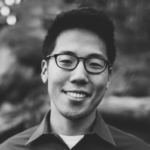
Databricks Compute Comparison: Classic Jobs vs Serverless Jobs vs SQL Warehouses