GRADIENT
AI compute optimization
Reduce cloud costs, increase engineering time and consistently meet your runtime SLAs with Gradient by Sync.
Get started in 3 easy steps:
Monitor
jobs
Gain actionable insights into job costs and performance, as we collect the statistical information required for accurate projections.
Optimize
clusters
Instantly identify opportunities for optimizations, as models are fine-tuned to each job to offer 100% customized optimizations.
Autopilot
for scalability
Review recommendations and apply them in a click, or enable auto-apply for scalability when you have 100s of jobs.
Effortlessly optimize
your Databricks ecosystem
Spun out of MIT, Gradient uses the advanced machine-learning algorithms to help organizations reduce spend and meet their SLAs.
Automatic optimization 24/7
- Continuous monitoring & optimization
- Adaptive to varying data sizes
- Lowers costs while obeying SLAs
- Saves 10 hours/ week per engineer
- Co-pilot and autopilot modes
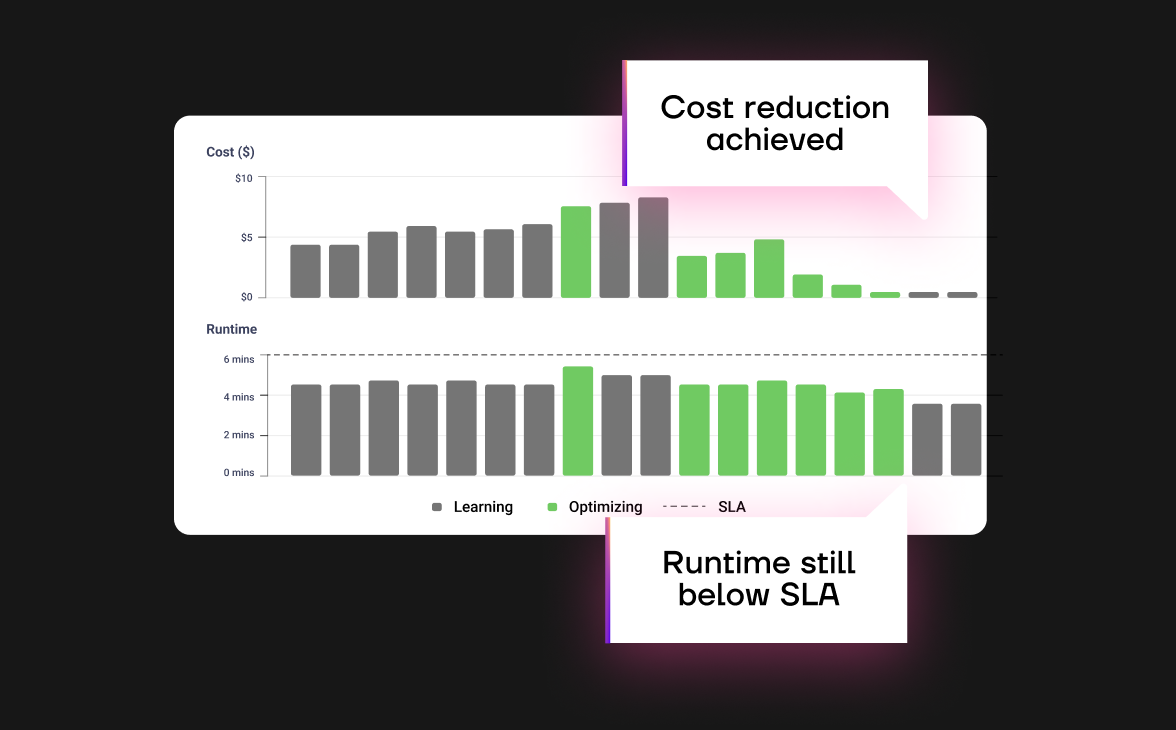
Custom tuned ML algorithms
- Automatically fine-tuned algorithms
- Self improving models via a closed-loop feedback
- The core model is based on historical performance logs
- Each job has a custom trained optimization model
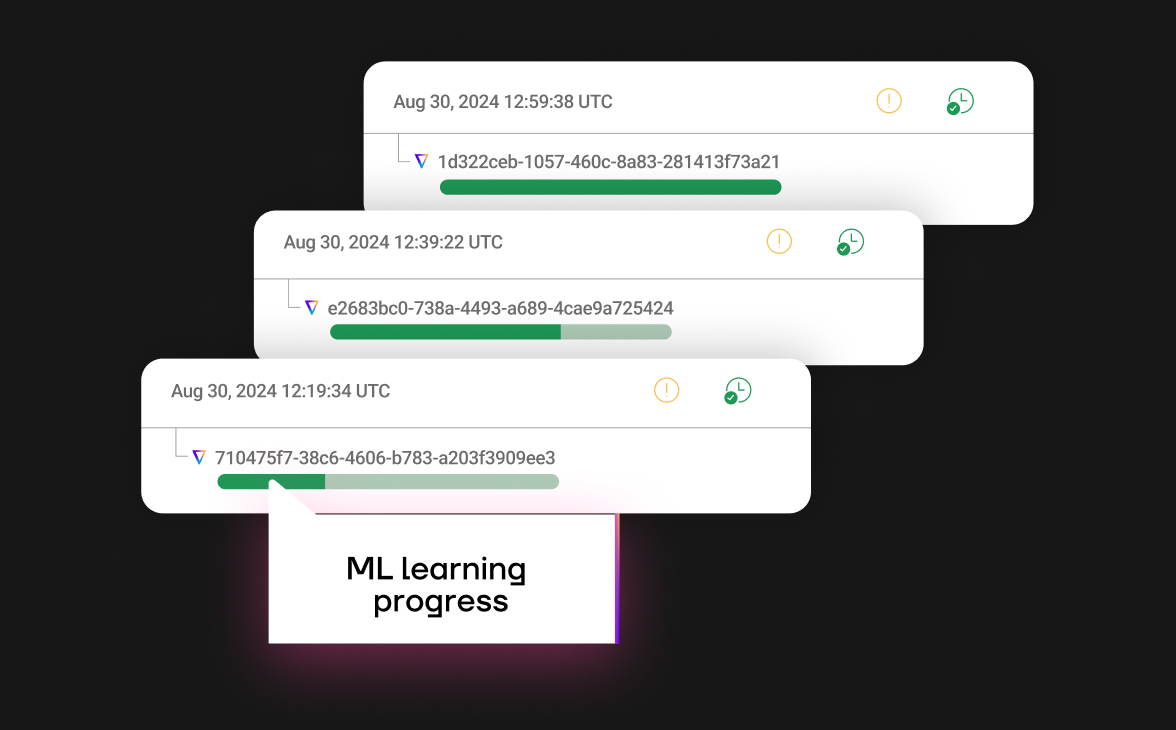
Control plane for your data
- Single pane of glass for your Databricks Workspace
- Total spend, total cost savings and ROI data
- Costs per job, customer, app-id, and custom tag
- Detailed recommendations for you to approve, or auto-apply
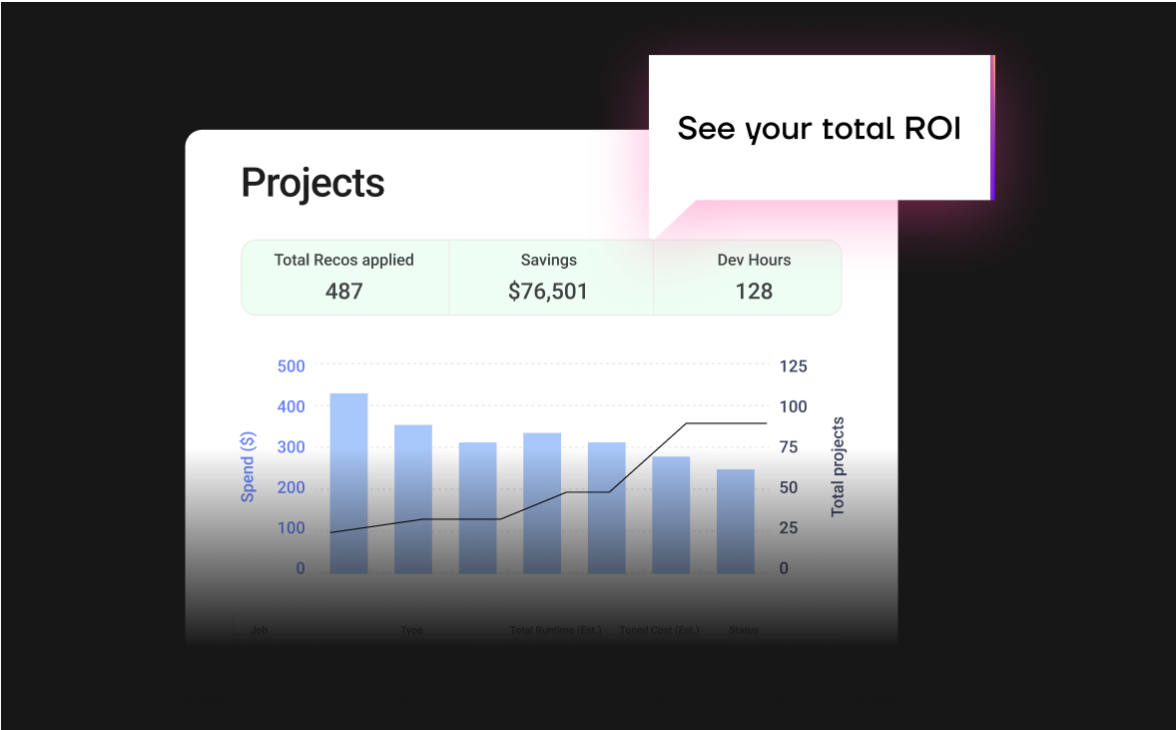
Complex data pipelines
- Adapts to cyclic patterns, seasonality, data spikes, and other complex patterns.
- Directed Acyclic Graph (DAG) dependent workflows
- Parallel jobs running on multiple nodes
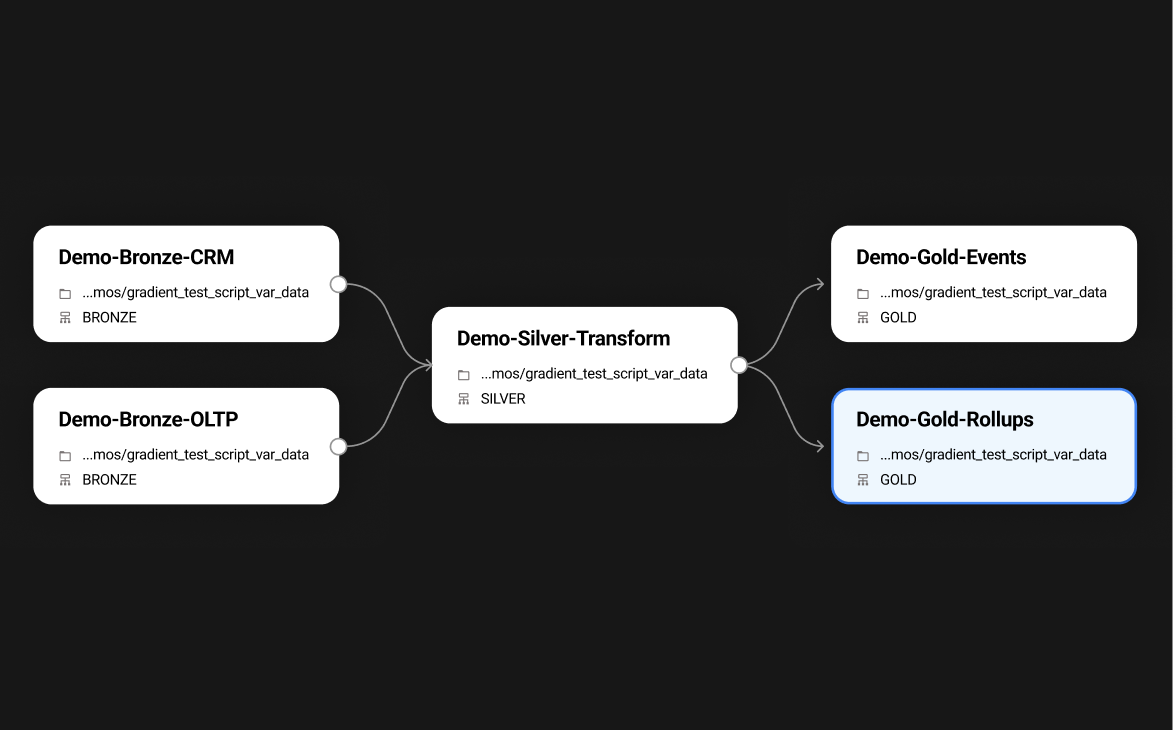
The benefits of serverless
without the drawbacks
- The convenience of serverless, with control
- Better cost optimization by 60%
- Retain full control over your jobs and clusters
- Full transparency on what the infrastructure is doing
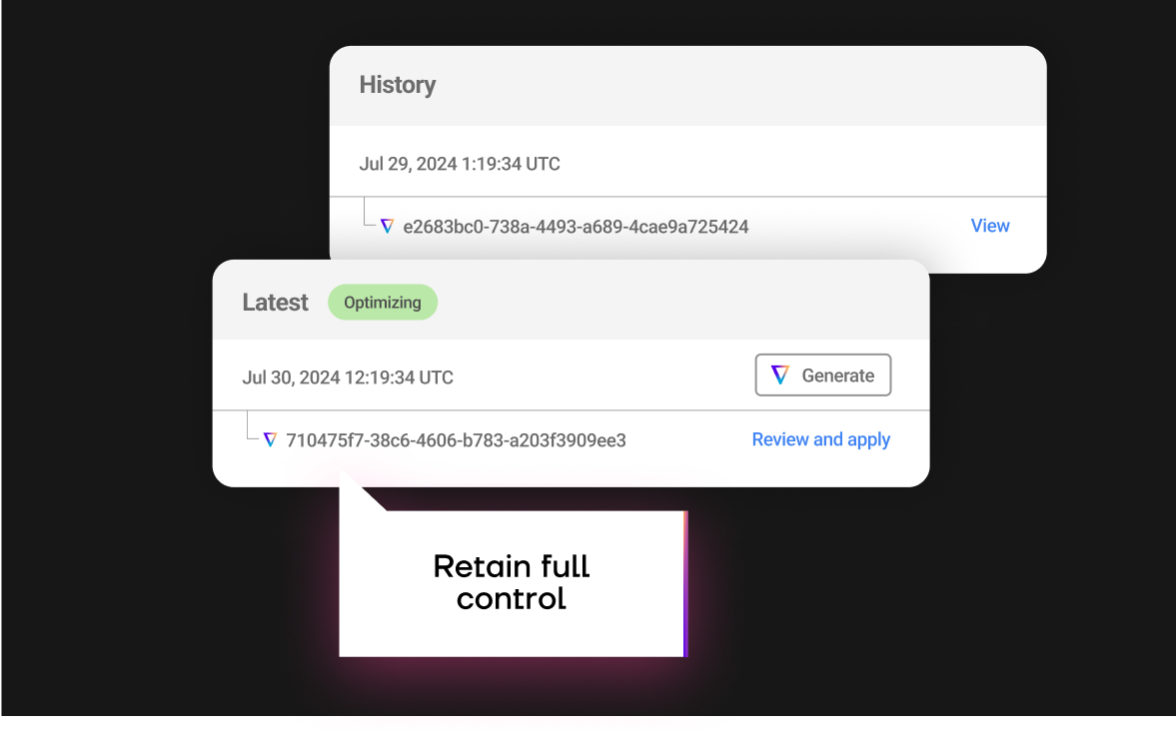
Compute cluster
optimization at scale
- AI built to optimize jobs at scale
- A single engineer can optimize 1000s of jobs
- A true “set and forget” solution
- Saves each data engineer 10 hours a week
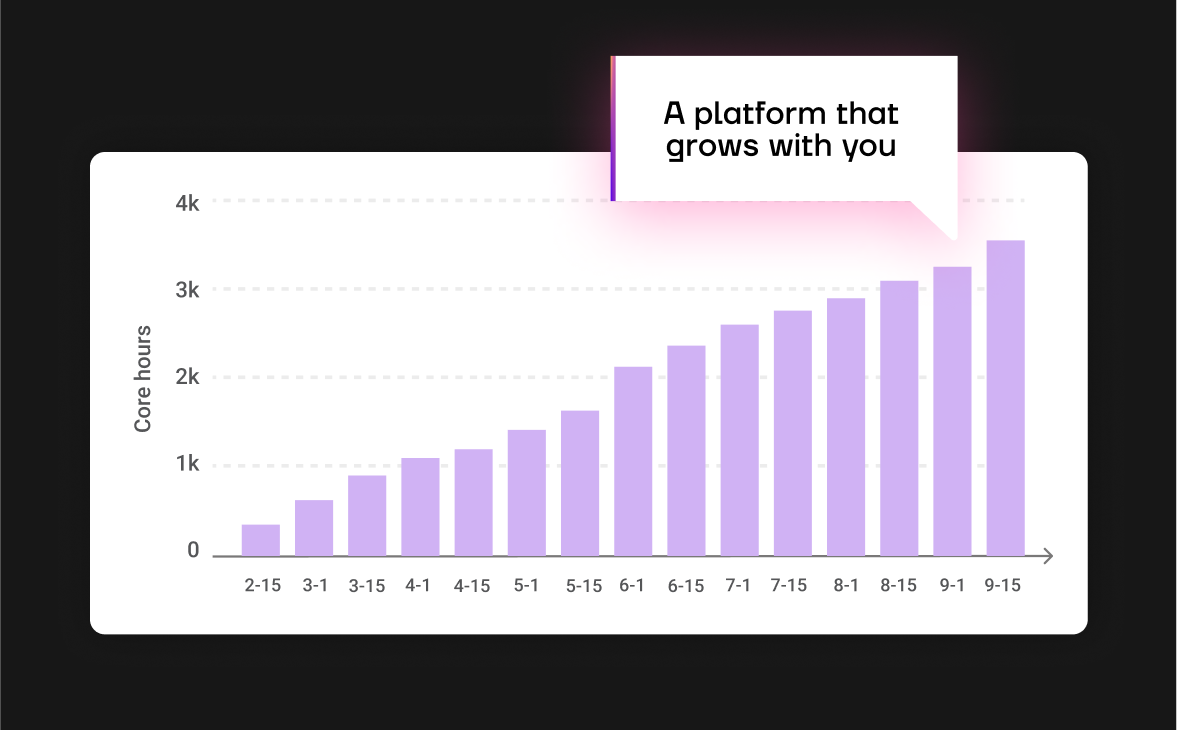
Root cause analysis
- Easy detection of root causes for runtime and cost anomalies
- Detailed recommendations logs
- Automated audit trails for every change made
- One click rollbacks
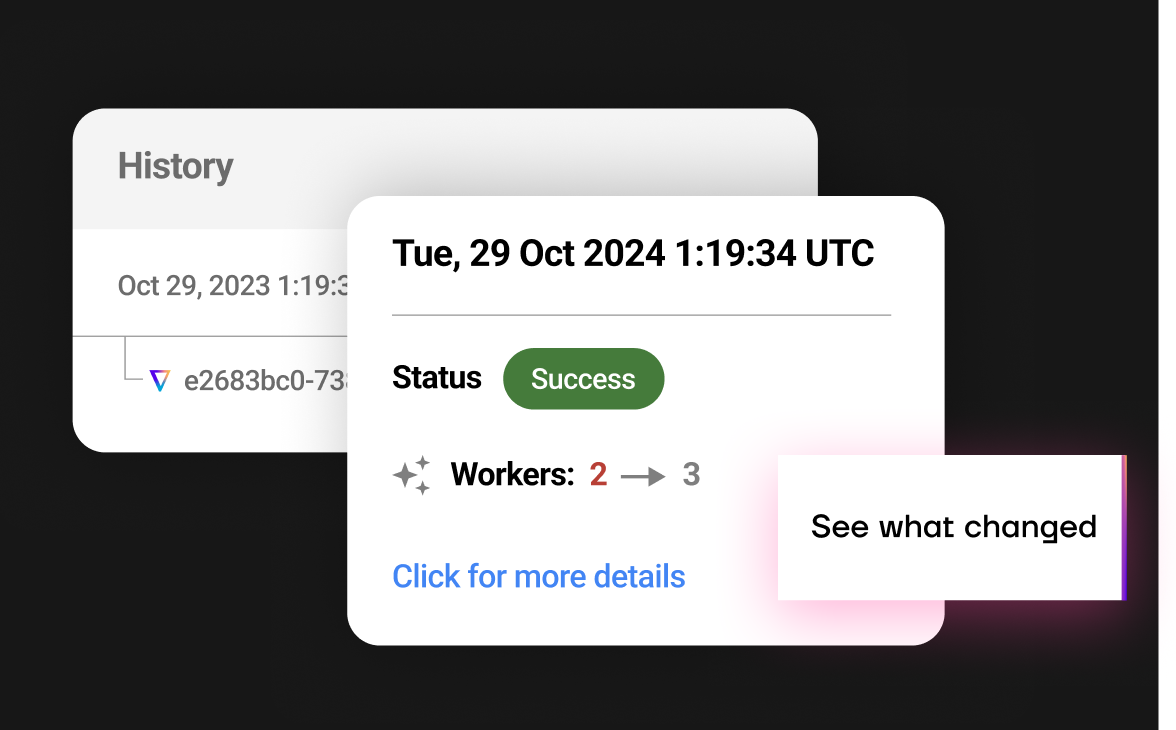
Fine-grained reports
- Spark metrics timelines to understand changes over time
- Performance by core hours, number of workers, input size, Spark shuffling, and more
- Job-level spend on DBUs and cloud costs
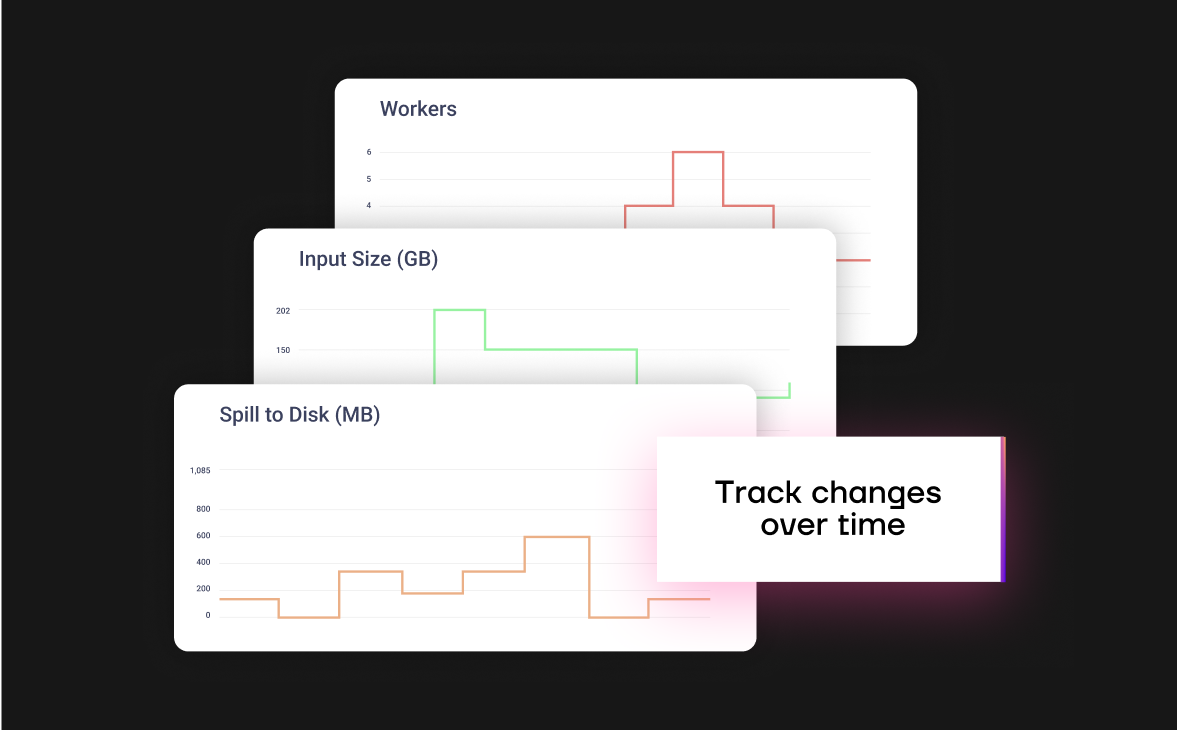
Auto-apply for
self-improving jobs
- Recommendations are automatically applied after each run
- Improvements through a closed feedback loop
- See the changes take effect by running the auto-training notebook
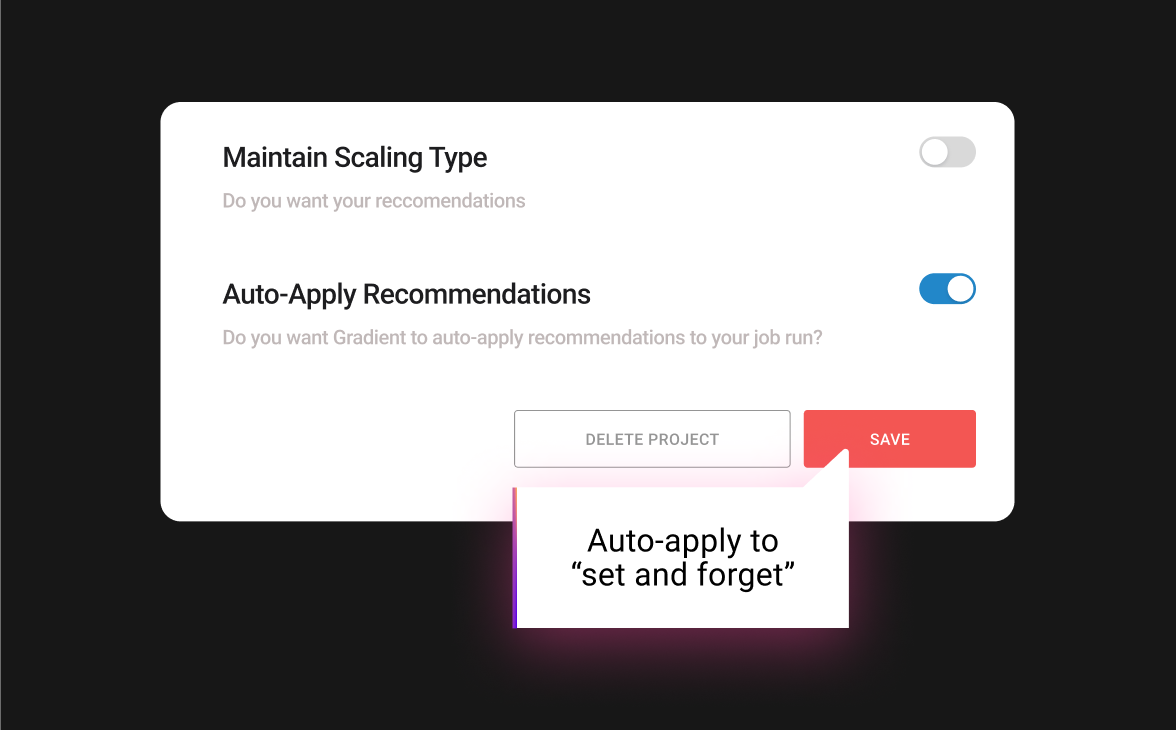
Works with
your stack
- Compatible with AWS and Azure (GCP soon)
- Airflow and Databricks Workflows integrations
- Azure Data Factory, Dagster, and others via API
- Custom integrations via API
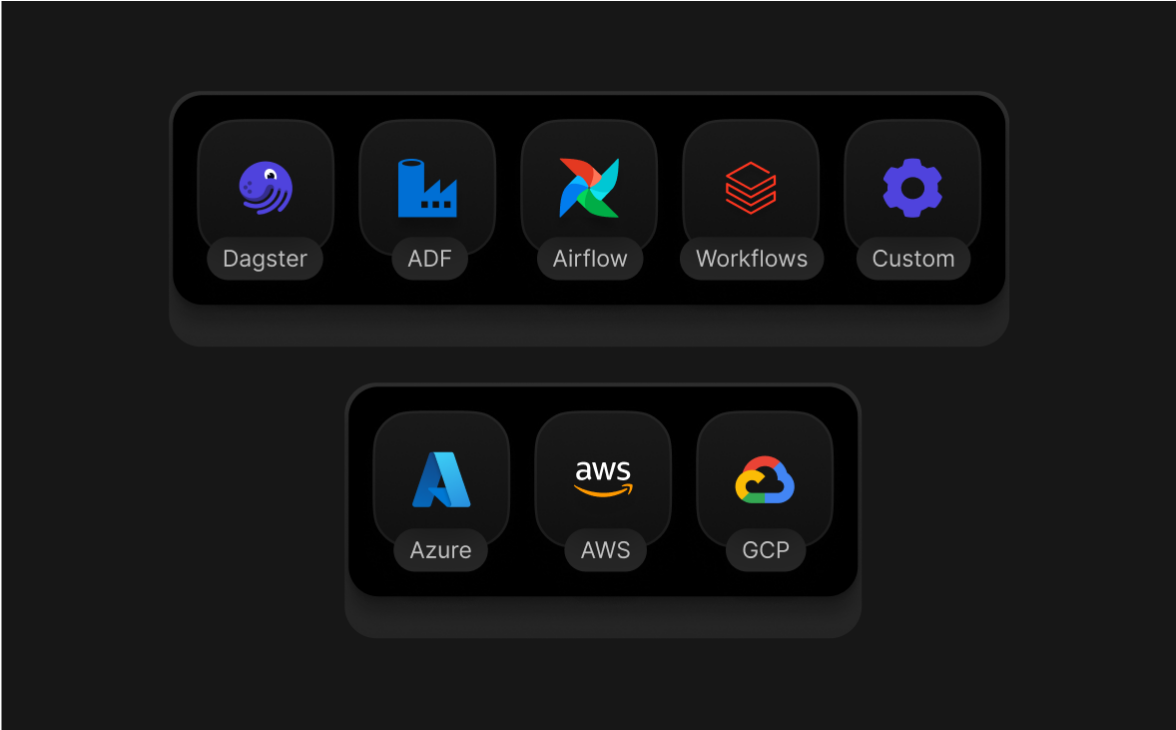
How it works
Get to know the sophisticated technology behind the world’s first machine learning compute optimization engine.

Data-driven model
Our core model collects statistical data about your jobs to train and determine the most effective and cost-efficient configuration to meet your desired outcomes (e.g. cost, runtime, or SLAs achievement rate).

Job-specific models
Every job has a model that is trained on worker numbers, cost, and data size. Gradient uses this information to construct the scaling curve per job to ensure accuracy.

Closed-loop feedback
Models are fine-tuned using a closed feedback loop where the optimizations are made and their impact on costs and performance is analyzed. This results in models that are automatically customized to your workloads.
Start saving in minutes
Schedule a call to go over your specific use-case
and learn how Gradient can help.