5 Lessons learned from testing Databricks SQL Serverless + DBT
We ran a $12K experiment to test the cost and performance of Serverless warehouses and dbt concurrent threads, and obtained unexpected results.
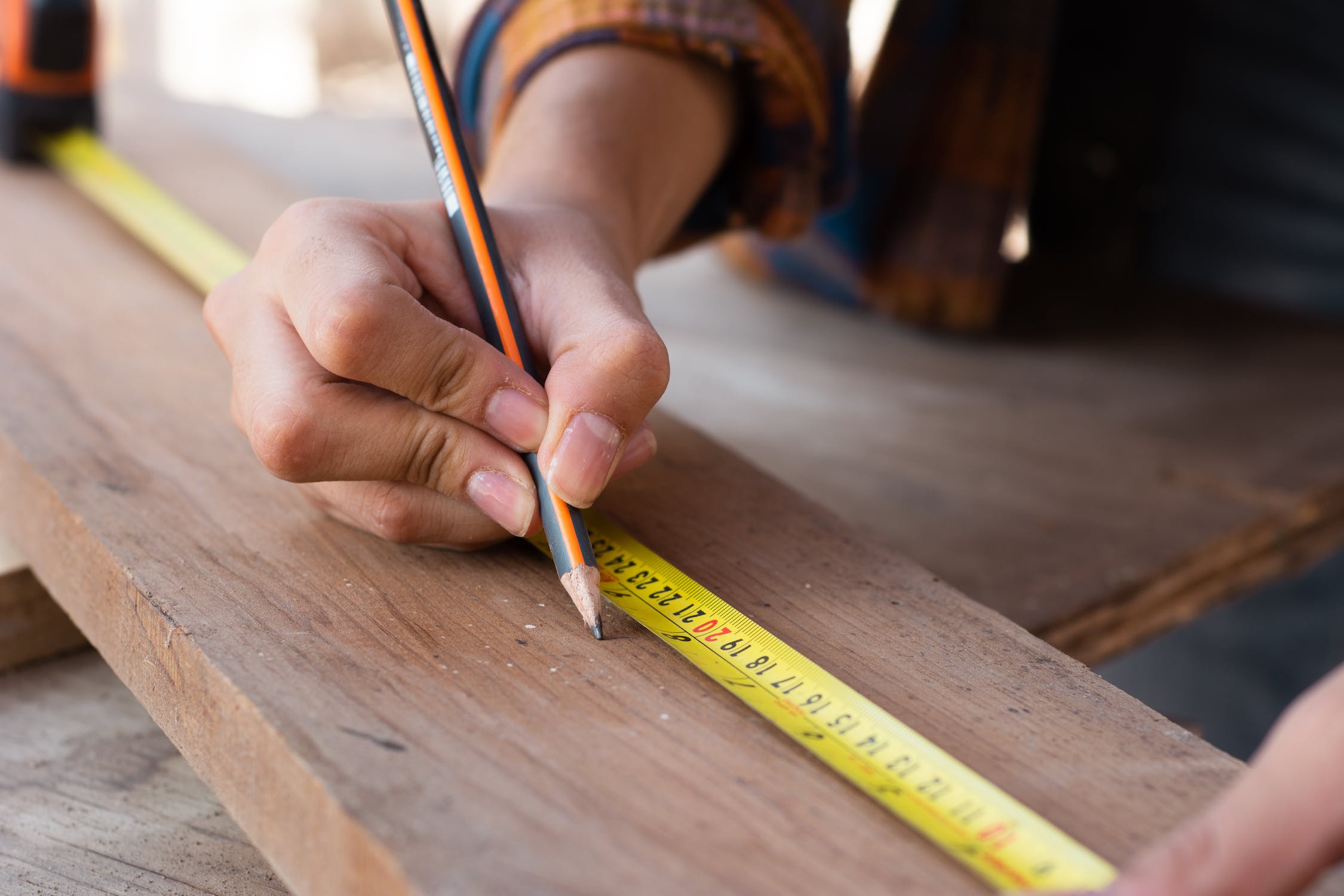
Databricks’ SQL warehouse products are a compelling offering for companies looking to streamline their production SQL queries. However, as usage scales up, the cost and performance of these systems become crucial to analyze.
In this blog we take a technical deep dive into the cost and performance of their serverless SQL warehouse product by utilizing the industry standard TPC-DI benchmark. We hope data engineers and data platform managers can use the data presented here to make better decisions when it comes to their data infrastructure choices.
What are Databricks’ SQL warehouse offerings?
Before we dive into a specific product, let’s take a step back and look at the different options available today. Databricks currently offers 3 different warehouse options:
- SQL Classic – Most basic warehouse, runs inside customer’s cloud environment
- SQL Pro – Improved performance and good for exploratory data science, runs inside customer’s cloud environment
- SQL Serverless – “Best” performance, and the compute is fully managed by Databricks.
From a cost perspective, both classic and pro run inside the user’s cloud environment. What this means is you will get 2 bills for your databricks usage – one is your pure Databricks cost (DBU’s) and the other is from your cloud provider (e.g. AWS EC2 bill).
To really understand the cost comparison, let’s just look at an example cost breakdown of running on a Small warehouse based on their reported instance types:
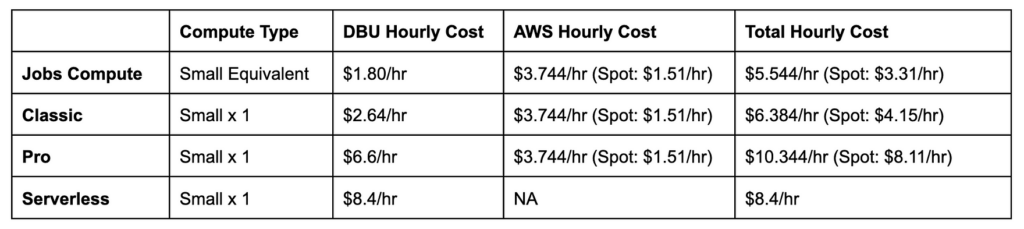
In the table above, we look at the cost comparison of on-demand vs. spot costs as well. You can see from the table that the Serverless option has no cloud component, because it’s all managed by Databricks.
Serverless could be cost effective compared to pro, if you were using all on-demand instances. But if there are cheap spot nodes available, then Pro may be cheaper. Overall, the pricing for serverless is pretty reasonable in my opinion since it also includes the cloud costs.
We also included the equivalent jobs compute cluster, which is the cheapest option across the board. If cost is a concern to you, you can run SQL queries in jobs compute as well!
Pros and cons of Serverless
The Databricks serverless option is a fully managed compute platform. This is pretty much identical to how Snowflake runs, where all of the compute details are hidden from users. At a high level there are pros and cons to this:
Pros:
- You don’t have to think about instances or configurations
- Spin up time is much less than starting up a cluster from scratch (5-10 seconds from our observations)
Cons:
- Enterprises may have security issues with all of the compute running inside of Databricks
- Enterprises may not be able to leverage their cloud contracts which may have special discounts on specific instances
- No ability to optimize the cluster, so you don’t know if the instances and configurations picked by Databricks are actually good for your job
- The compute is a black box – users have no idea what is going on or what changes Databricks is implementing underneath the hood.
Because of the inherent black box nature of serverless, we were curious to explore the various tunable parameters people do still have and their impact on performance. So let’s drive into what we explored:
Experiment Setup
We tried to take a “practical” approach to this study, and simulate what a real company might do when they want to run a SQL warehouse. Since DBT is such a popular tool in the modern data stack, we decided to look at 2 parameters to sweep and evaluate:
- Warehouse size – [‘2X-Small’, ‘X-Small’, ‘Small’, ‘Medium’, ‘Large’, ‘X-Large’, ‘2X-Large’, ‘3X-Large’, ‘4X-Large’]
- DBT Threads – [‘4’, ‘8’, ’16’, ’24’, ’32’, ’40’, ’48’]
The reason why we picked these two is they are both “universal” tuning parameters for any workload, and they both impact the compute side of the job. DBT threads in particular effectively tune the parallelism of your job as it runs through your DAG.
The workload we selected is the popular TPC-DI benchmark, with a scale factor of 1000. This workload in particular is interesting because it’s actually an entire pipeline which mimics more real-world data workloads. For example, a screenshot of our DBT DAG is below, as you can see it’s quite complicated and changing the number of DBT threads could have an impact here.
As a side note, Databricks has a fantastic open source repo that will help quickly set up the TPC-DI benchmark within Databricks entirely. (We did not use this since we are running with DBT).
To get into the weeds of how we ran the experiment, we used Databricks Workflows with a Task Type of dbt as the “runner” for the dbt CLI, and all the jobs were executed concurrently; there should be no variance due to unknown environmental conditions on the Databricks side.
Each job spun up a new SQL warehouse and tore it down afterwards, and ran in unique schemas in the same Unity Catalog. We used the Elementary dbt package to collect the execution results and ran a Python notebook at the end of each run to collect those metrics into a centralized schema.
Costs were extracted via Databricks System Tables, specifically those for Billable Usage.
Try this experiment yourself and clone the Github repo here
Results
Below are the cost and runtime vs. warehouse size graphs. We can see below that the runtime stops scaling when you get the medium sized warehouses. Anything larger than a medium pretty much had no impact on runtime (or perhaps were worse). This is a typical scaling trend which shows that scaling cluster size is not infinite, they always have some point at which adding more compute provides diminishing returns.
For the CS enthusiasts out there, this is just the fundamental CS principal – Amdahls Law.
One unusual observation is that the medium warehouse outperformed the next 3 sizes up (large to 2xlarge). We repeated this particular data point a few times, and obtained consistent results so it is not a strange fluke. Because of the black box nature of serverless, we unfortunately don’t know what’s going on under the hood and are unable to give an explanation.
Runtime in Minutes across Warehouse Sizes.
Because scaling stops at medium, we can see in the cost graph below that the costs start to skyrocket after the medium warehouse size, because well basically you’re throwing more expensive machines while the runtime remains constant. So, you’re paying for extra horsepower with zero benefit.
Costs across Warehouse Sizes.
The graph below shows the relative change in runtime as we change the number of threads and warehouse size. For values greater than the zero horizontal line, the runtime increased (a bad thing).
The data here is a bit noisy, but there are some interesting insights based on the size of the warehouse:
- 2x-small – Increasing the number of threads usually made the job run longer.
- X-small to large – Increasing the number of threads usually helped make the job run about 10% faster, although the gains were pretty flat so continuing to increase thread count had no value.
- 2x-large – There was an actual optimal number of threads, which was 24, as seen in the clear parabolic line
- 3x-large – had a very unusual spike in runtime with a thread count of 8, why? No clue.
The Percent Change in Runtime as Threads Increase.
To put everything together into one comprehensive plot, we can see the plot below which plots the cost vs. duration of the total job. The different colors represent the different warehouse sizes, and the size of the bubbles are the number of DBT threads.
Cost vs duration of the jobs. Size of the bubbles represents the number of threads. Image by author
In the plot above we see the typical trend that larger warehouses typically lead to shorter durations but higher costs. However, we do spot a few unusual points:
- Medium is the best – From a pure cost and runtime perspective, medium is the best warehouse to choose
- Impact of DBT threads – For the smaller warehouses, changing the number of threads appeared to have changed the duration by about +/- 10%, but not the cost much. For larger warehouses, the number of threads impacted both cost and runtime quite significantly.
Conclusion
In summary, our top 5 lessons learned about Databricks SQL serverless + DBT products are:
- Rules of thumbs are bad – We cannot simply rely on “rules of thumb” about warehouse size or the number of dbt threads. Some expected trends do exist, but they are not consistent or predictable and it is entirely dependent on your workload and data.
- Huge variance – The costs ranged from $5 – $45, and runtimes from 2 minutes to 90 minutes, all due to different combinations of number of threads and warehouse size.
- Serverless scaling has limits – Serverless warehouses do not scale infinitely and eventually larger warehouses will cease to provide any speedup and only end up causing increased costs with no benefit.
- Medium is great – We found the Medium Serverless SQL Warehouse outperformed many of the larger warehouse sizes on both cost and job duration for the TPC-DI benchmark. We have no clue why.
- Jobs clusters may be cheapest – If costs are a concern, switching to just standard jobs compute with notebooks may be substantially cheaper
The results reported here reveal that the performance of black box “serverless” systems can result in some unusual anomalies. Since it’s all behind Databrick’s walls, we have no idea what is happening. Perhaps it’s all running on giant Spark on Kubernetes clusters, maybe they have special deals with Amazon on certain instances? Either way, the unpredictable nature makes controlling cost and performance tricky.
Because each workload is unique across so many dimensions, we can’t rely on “rules of thumb”, or costly experiments that are only true for a workload in its current state. The more chaotic nature of serverless system does beg the question if these systems need a closed loop control system to keep them at bay?
As an introspective note – the business model of serverless is truly compelling. Assuming Databricks is a rational business and does not want to decrease their revenue, and they want to lower their costs, one must ask the question: “Is Databricks incentivized to improve the compute under the hood?:
The problem is this – if they make serverless 2x faster, then all of sudden their revenue from serverless drops by 50% – that’s a very bad day. If they could make it 2x faster, and then increase the DBU costs by 2x to counteract the speedup, then they would remain revenue neutral (this is what they did for Photon actually).
So Databricks is really incentivized to decrease their internal costs while keeping customer runtimes more or less the same. While this is great for Databricks, it’s difficult to pass on any acceleration technology to the user that results in a cost reduction.
Interested in learning more about how to improve your Databricks pipelines? Reach out to Jeff Chou and the rest of the Sync Team.
Resources
Related Content
More from Sync:
Sync’s Health Check for Databricks Workspaces
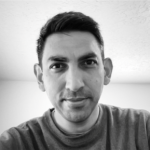
Sync’s Health Check for Databricks Workspaces
Why are your Databricks jobs performances changing over time?
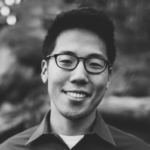
Why are your Databricks jobs performances changing over time?